by Hugo Schnack
Magnetic resonance imaging (MRI) is a very useful tool for in vivo detection of possible morphological differences in the brains of psychiatric patients as compared to healthy persons. Due to the relatively large size of the voxels - the 3D pixels that make up the images - classification is difficult. Simulated 'orange-in-a-box' images can help to improve the classification algorithms.
The brains of psychiatric patients may be different from those of healthy people in size and shape; this could be a cause or a result of the disease. One way to investigate this is making MRI brain scans of patients and control subjects. The information in the images is quantified by segmenting them. The voxels - the building blocks of the images - are attributed to different tissue classes. The three main brain-tissue types of interest are grey matter (neurons, mainly in the cortical surface), white matter (connecting fibres), and cerebrospinal fluid (CSF). The latter is not a real tissue: rather it is the fluid surrounding the brain and filling several holes in the brain (see Figure 1, left and middle). The cortical surface is thin (~ 2mm) and highly folded, and therefore most voxels (of size ~ 1mm3) that sample the cortex are only partly filled with grey matter, with the rest being CSF or white matter. These voxels have a brightness somewhere between the brightnesses of the pure tissues, and this so-called partial-volume effect hinders a straightforward classification of MR brain images. The reason for this is that segmentation algorithms must simultaneously estimate intrinsic tissue parameters (means and variances of their brightness distributions) and tissue volume fractions of the voxels, depending on the morphology of the brain.
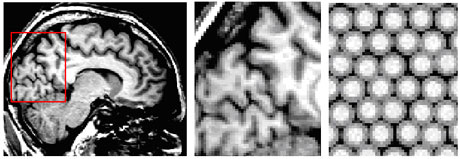
An MRI brain tissue segmentation algorithm should produce reliable estimates of the tissue distributions and volumes. In particular, for analysis of images from patients and control subjects, the algorithm should be unbiased in classification of images of brains with different morphology.
Our goal was to test the robustness of our segmentation algorithm: how much influence does the structure of the brain have on the estimation of the intrinsic tissue properties, ie the intensity means? Since the true tissue distributions of real MR brain images are unknown, we designed a model that simulates images with three tissues and with different structural organizations, mimicking the relevant properties of the natural situation as much as possible. The brain tissue is modelled as a collection of white matter spheres with a grey matter peel (the cortex): "oranges". The oranges are placed in a sea of CSF (see right picture of the figure). The parameters to be varied are the size of the white matter cores and the grey matter peel thicknesses, reflecting brain size and cortical thickness respectively. Furthermore the distances between the oranges can be varied from densely packed to not touching at all, the latter reflecting atrophy. Some randomness is added to each oranges parameter value in order to model the variation of cortical properties throughout the brain. Finally, noise is added to the image. Any number of oranges can be created.
We used these images of oranges to test our tissue classification algorithm. The algorithm analyses the intensity histograms of the images and gives probabilistic segments, ie for each voxel a probability of being grey matter, white matter, or CSF is calculated.
First we ran our classification algorithm on several simulated images to test if it could find within a certain precision the simulated intrinsic tissue parameters. This was successful, and the calculated tissue volumes were equal to the modelled volumes within 1-2%. Then we ran our algorithm on a series of simulations in which we varied the average packing distance or peel thickness in a regular way, creating small to large, but realistic, numbers of partial-volume voxels, in order to test the influence of the structure on the classification results. The influence turned out to be minimal - about 1% of the mean grey matter intensity and 3% of the grey/white contrast for a wide range of distances.
In conclusion, although MR brain images and our oranges-in-a-box appear quite different, the latter are in fact surprisingly suitable as realistic test images for tissue classification algorithms.
This research project was carried out by Hugo Schnack and Rachel Brouwer of the neuroimaging section of the Rudolf Magnus Institute of Neuroscience. This is located in the Department of Psychiatry at the University Medical Center Utrecht, in the Netherlands.
Link:
http://www.smri.nl
Please contact:
Hugo Schnack
University Medical Center Utrecht, the Netherlands
Tel.: +31 30 2508459
E-mail: hschnackazu.nl