by Matej Oresič, Jyrki Lötjönen and Catherine Bounsaythip
There has long been a consensus that there is a pressing need to bridge the gap between basic and clinical sciences, to ensure that basic research discoveries of potential relevance to patient care are effectively applied. This is a formidable challenge to implement. One of the key problems is the lack of a framework or model that would link clinically relevant information to the knowledge obtained across multiple disciplines, experimental platforms and biological systems.
The overall objective of our project is to develop a comprehensive visualization and modelling framework to enable a multi-level integration of biological and clinical data. The primary focus areas are:
-
multi-level biomedical data integration using a conceptual space approach
-
linking medical image data with molecular pathway level information
-
cross-species phenotype mappings and translational biomarkers.
This project gathers people across several domains at VTT, including systems biology, signal processing, medical imaging, data mining and software engineering. The research has been performed in close cooperation with medical experts. The conceptual space approach was developed in collaboration with the Computational Cognitive Systems group at Helsinki University of Technology.
Beyond the current Semantic Web: A Novel Conceptual Approach to Tackling the Complexity of Knowledge Representation
Most current approaches to life science data integration are conceptually based on methods that were developed when information was scarce. With the pace at which data volumes are increasing, these approaches face the challenge of evolving concepts and context sensitivity. For a knowledge model to be adaptive, it must support emergence of new concepts and knowledge structures in a context-specific manner.
Conceptual spaces have recently emerged as a flexible framework to tackle the problem of context-based concept formation and evolution. The theory of conceptual spaces combines elements from other theories in cognitive science, psychology and linguistics. It is based on the topological analysis of the information space that enables similarity to be modelled and computed in a natural way, using appropriate metrics. The information space can embed many other spaces, which makes the paradigm suitable for tackling the problem of multi-scale data integration in systems biology.
Metabolome: Sensitive Readout of Human Physiology
Metabolomics has recently emerged as one of the key platforms for medical systems biology and translational research. Patterns of metabolites (small molecules) in biofluids and tissues reflect the homeostasis of the organism. The human metabolome is affected by factors such as lifestyle, nutrition and gut microbiota, which are of particular relevance to complex diseases believed to be due to interactions between genetic factors and the environment. Metabolites are also common across species, unlike other levels of molecular biology, and hence might represent the best chance of cross-species biomarkers.
We applied the metabolomics strategy in multiple clinical and preclinical studies. The metabolic profiles obtained in these studies contain valuable information about clinical phenotypes, which can be utilized as a link between the human physiological level and local alterations of molecular pathways.
Integrating Pathways and Medical Images
The conceptual space framework is well suited to the integration with molecular-level information of complex clinical data such as medical images. The software tool that we have developed, megNET®, implements the conceptual space approach for mining and visualizing life science and medical data by utilizing state-of-the-art 3D techniques, mathematical modelling techniques, and contextualization (see Figure).
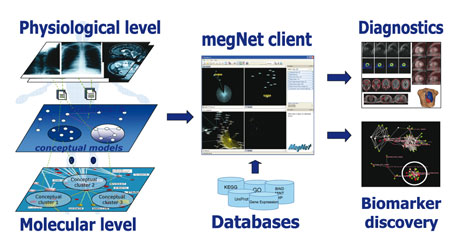
The essential part of our data integration strategy is the highly automated and accurate image quantification accomplished by our image analysis tool. Statistical models can thus be developed to cluster disease-related phenotypes based on image data, as has already been performed using molecular profiling techniques. Mappings between the medical image level and molecular profiles and networks can be established in two ways: either based on statistical models, which are optimal if data at multiple levels is available from the same individuals, or based on matching the clinical annotations using biomedical ontologies.
As a case study, we have been collecting a large number of cardiac magnetic resonance images and other clinical data related to dilated cardiomyopathy caused by Lamin A/C mutation. Serum samples from a clinical trial have been collected from the same individuals for metabolomics analyses. This data is complemented by the establishment of molecular networks based on published microarray data related to the topic, as well as by the integration of relevant molecular interaction networks using the megNet® environment. In a typical megNet® query, the user inputs biological entities and concepts, such as "Lipoxygenase AND Lamin A/C mutation AND females", from which a network of relations in clinical and biological databases is built and visualized.
Cross-Species Mapping for Translational Medicine: Type 1 Diabetes Pathogenesis and Prediction
Type 1 diabetes (T1D) is the most prominent metabolic-endocrine disease among children in the western world. Since 2005 we have been involved in the Finnish Type 1 Diabetes Prediction and Prevention Study (DIPP), a large birth cohort study, in order to identify novel molecular markers that characterize the development of diabetes-associated autoimmunity and progression towards overt clinical T1D.
Much of the current knowledge on T1D was obtained using preclinical models, and establishing the direct clinical relevance of these findings has been difficult. Not surprisingly, over one hundred therapies successfully tested in preclinical models have so far proved unsuccessful in a clinical setting, and at present there is still no cure for the disease.
This problem of translation of knowledge from preclinical models to successful therapies is one of the key bottlenecks in today's pharmaceutical pipelines. We addressed this challenge by initiating a project called "In silico models of disease pathogenesis and therapy (TRANSCENDO)." The objective of the project is to generate a translational biomarker bridge between the large-scale molecular profiling in a clinical setting to molecular profiles obtained in a preclinical setting, with the primary focus on T1D. The model will enable us to link knowledge on molecular pathways related to T1D pathogenesis, as well as to develop and test new therapies for disease prevention and treatment.
While the TRANSCENDO strategy provides a methodology for a comprehensive translational medicine implementation, it also addresses the issue of a true systemic integration of cell-, tissue-, or cross-organ-specific information, including molecular pathways. The megNet® environment has been used to enrich our statistical model based on longitudinal molecular profiles in clinical and preclinical settings, with vast amounts of information on molecular pathways and physiology.
Perspectives
Our conceptual space strategy, implemented using the megNet® environment, has already demonstrated its potential in clinical applications. We believe that our approach will be very useful in building complex in silico models at the level of human physiology, making mappings across multiple levels of biological organization and across multiple knowledge domains a feasible task.
One of the emergent challenges in life science and medical knowledge management is how to deal with the dynamics in biological systems, that is, how to encode the inherent dynamic properties of biological systems for the purpose of data mining and modelling. It is obvious that modelling at all levels, from quantum processes to physiology and environment, is computationally unfeasible. We believe that the conceptual spaces approach could help in establishing the relevant components of the system to be included in the models.
Since conceptual spaces are a powerful approach to build metaphors across different knowledge domains, one could also envision the applications of the approach outside the life science domain. We have recently initiated one such project, aiming to use agent-based modelling of biological cells in order to develop more flexible computing tools.
Links:
Quantitative Biology and Bioinformatics group at VTT: http://sysbio.vtt.fi/
VISUBIOMED project: http://sysbio.vtt.fi/visubiomed/
TRANSCENDO project: http://sysbio.vtt.fi/transcendo/
SYSDIPP project: http://sysbio.vtt.fi/sysdipp/
Helsinki University of Technology, Laboratory of Computer and Information Science: http://www.cis.hut.fi/
Please contact:
Matej Oresič
VTT Technical Research Centre of Finland
Tel: +358 20 722 4491
E-mail: matej.oresicvtt.fi