by Filippo Geraci, Mauro Leoncini, Manuela Montangero, Marco Pellegrini and Maria Elena Renda
A new approach to the analysis of large data sets resulting from microarray experiments yields high-quality results that are orders of magnitude faster than competing state-of-the-art approaches. This overcomes a significant performance bottleneck normally evident in such complex systems.
Modern personalized medicine relies heavily on molecular analysis and imaging, and so requires a range of support systems that include integrated health information systems, digital models for personalized simulations, and advanced diagnostic systems. Technology is of greatest use to decision makers if it is able to produce clear and useful indications in an accurate and timely manner.
Advances in microarray technology have reached a stage where it is possible to "watch" simultaneously the activation state of all the genes of a given biological entity under a variety of external stimuli (drugs, diseases, toxins etc). The analysis of the gene expression data complements the better-known analysis of the individual variations in the genetic code.
To use a computer programming analogy, in order to understand the workings of a piece of code in a computer, it is often necessary to look at internal changes during execution, rather than just looking at the program as an isolated static piece of text. This is even truer in biology since it is by now clear that the genetic code, though very important, is just one ingredient in a far more complex biological mechanism.
Our research fits neatly within the "Digital Human Modelling" initiative since it removes technical obstacles standing in the way of the personalization of digital models. Ideally we should strive for a different model for each individual human being. In practice we should collect as much information as possible related to a single human being, at different levels, so as to be able to fine-tune our existing generalist models. On the one hand, we are living in the "age of data", and existing technology is able to produce a deluge of data related to a single patient: data on organs, tissues, and as far down as the molecular level. On the other hand, a burden is placed on our data processing capabilities that is only partially alleviated by advances in hardware performance. Our aim is to identify and tackle algorithmic bottlenecks blocking the pipeline that connects data collection with useful simulation and diagnostics.
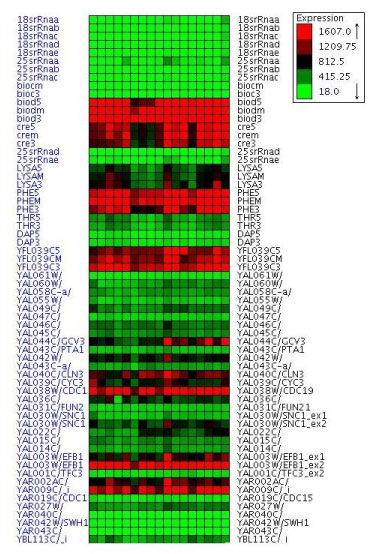
Gene expression data from a single microarray experiment can trace the activities of a number of genes ranging from a few thousands to hundreds of thousands under hundreds of stimuli. Moreover large laboratories of pharmaceutical companies already perform tens of thousands of experiments each year (eg research labs at Merck & Co, Inc undertook roughly forty thousand microarray experiments in 2006).
In order to extract useful information from this amount of data it is customary to apply the clustering unsupervised learning technique. This involves automatically grouping together genes with a similar expression profile. The human analyst then has the simplified task of checking a few dozen groups (clusters) of genes, instead of thousands of individual genes.
Unfortunately existing state-of-the-art clustering methodologies cannot cope with this data deluge. Some do not scale well to such large data sets, requiring hours or days of computation on powerful workstations. Others are unable to determine critical parameters automatically such as the optimal number of clusters, and thus rely on educated guesses or rote repetitions.
Our project is developing and demonstrating the effectiveness of a new class of clustering algorithms that are able to cope with massive data sets in a fraction of the time needed by current state-of-the-art techniques. In addition, these algorithms can retain or even improve the quality of the output, and can detect automatically the optimal number of clusters. We have already attained improvements in speed of up to a factor of ten to twenty on relatively small data sets of six thousand genes. We employ techniques from computational geometry developed for clustering points in metric spaces coupled with information theoretic optimality criteria.
In a parallel research activity, this methodology has been already successfully applied to information retrieval on textual data. Our approach has been validated by comparing our results with the well-known annotated gene list for yeast (Saccharomyces cerevisiae), maintained by the Gene Ontology Consortium.
Future activities involve the application of our techniques to specific medical problems related to the analysis of tumour growth. One of the most important discoveries in recent years has been that many types of cancer result from genomic changes acquired by somatic cells during their lifetime. Moreover, tumour growth evolves in a series of stages, each with characteristic features and metabolic mechanisms. Thus a complete description, modelling and prediction can be made only by collecting large amounts of data over time, at the molecular level (gene expressions), the tissue level (via medical imaging technology) and the clinical level. Current models of tumour growth will be greatly improved by the integration, correlation and cross-validation of molecular, imaging and clinical data.
This research is carried out in Pisa at the Institute for Informatics and Telematics of the Italian Research Council (IIT-CNR), by a team composed of researchers from IIT-CNR and the Department of Information Engineering of the University of Modena and Reggio Emilia. The activity began in 2006 as part of the CNR Bioinformatics Inter-Departmental Project. This research benefits greatly from the exchange of ideas taking place within the recently formed ERCIM Digital Patient Working Group.
Please contact:
Marco Pellegrini
IIT-CNR, Italy
Tel: +39 050 315 2410
E-mail: marco.pellegriniiit.cnr.it
http://www.iit.cnr.it/staff/marco.pellegrini/