by Angela Grassi
In the post-genomic era, identifying the structure of genetic networks is one of the main goals of Systems Biology. A project in which gene regulatory networks are modelled and reconstructed from time-course gene expression data is being undertaken by the Institute of Biomedical Engineering (ISIB-CNR) in collaboration with Lancaster University.
The behaviour of a living cell is regulated by complex networks of interaction between DNA, RNA, proteins and small molecules. With the availability of complete genome sequences and large-scale microarray data, the last fifteen years have seen a growing interest in the study of intracellular networks. Unravelling this complex organization is vital to obtaining a better understanding of normal and pathological cell physiology. In this work we focus on the so-called gene (or genetic) regulatory networks which describe the regulatory interactions at the gene level.
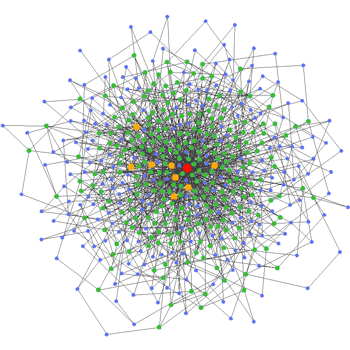
Genetic networks are usually visualized as directed graphs in which nodes represent genes and edges regulatory influences. In our model, edges are labeled with a sign (+ or -) indicating the nature of the regulation. The corresponding mathematical representation of gene interactions is the adjacency or gene interaction matrix, in which the i,j-th element may be either +1, -1 or 0: respectively, these designators mean that gene i activates, represses or does not regulate the expression of gene j.
Among the several approaches which have been proposed to infer the structure of gene regulatory networks, we decided to adopt one based on graphical models. Starting from time-course gene expression data, we construct a Bayesian hierarchical model that takes into account the biological knowledge about transcription, the process by which messenger RNA (mRNA) is copied from the genetic instructions contained in a gene. This process is regulated by proteins called transcription factors. We model the dynamics of transcription via nonlinear differential equations in which the protein levels of regulators are considered as unobserved parameters. Another important parameter of the model is the adjacency matrix whose choice takes into account the available biological knowledge.
Most biological networks tend to be organized according to some characteristic features: a relatively short path length between any two nodes (small world property), the presence of many genes with few connections and few highly connected genes (hubs), and the lethal impact for the overall architecture of the network of the deletion of a hub (centrality and lethality principle).
A particular class of networks that exhibits these features is the so-called scale-free class. The scale-free property means that the connectivity distribution (ie the probability distribution of the number of regulators of each gene) follows a power law.
Rather than inferring the topological structure from the data, we impose a scale-free topological constraint on the overall structure of the network choosing a power law for the connectivity distribution.
The identification of the model from real data is based on Markov Chain Monte Carlo (MCMC) techniques. The model is implemented via a hybrid Metropolis-Hastings and Gibbs sampler using the statistical software R. In the case of the adjacency matrix we use Approximate Bayesian computations, incorporating a frequentist testing strategy in the MCMC update.
The idea of imposing a Bayesian topological constraint on the overall structure of the network has already been used in a linear model framework. The novelty of our model resides in the use of a nonlinear model for transcription, which seems appropriate to better exploit the biological knowledge about this process. Moreover in our dynamics of transcription we take into consideration that a gene could have several regulators which could be either repressors or activators.
The completion of the project includes the refinement of the R code for the MCMC implementation, and its application to a real dataset. Future activities will be devoted to the extension of our model, an investigation of different types of topological constraints and the introduction of biological knowledge about translation, the process by which mRNA is translated into proteins.
This work is the result of collaboration with Ernst Wit, head of the Medical Statistics Unit at the Department of Mathematics and Statistics, Lancaster University. Angela Grassi is supported by a grant of Regione Veneto (Azione Biotech II - DGR 2112/02-08-05) to ISIB.
Links:
http://biotech.pd.cnr.it/
Please contact:
Angela Grassi
ISIB-CNR, Padova, Italy
Tel: +39 049 829 5752
E-mail: angela.grassiisib.cnr.it