by Emanuele Salerno and Simon Wilson
An invited session on computational learning methods for unsupervised segmentation was held on 14 September in the framework of the 11th International Conference on Knowledge-Based and Intelligent Information & Engineering Systems (KES 2007) in Vietri sul Mare, Italy. This initiative was taken by the MUSCLE 'e-team' on unsupervised segmentation and classification of multichannel data. MUSCLE (Multimedia Understanding through Semantics, Computation and Learning) is a European Network of Excellence managed by ERCIM.
Many measurement procedures are intended to extract information on physical systems by exploiting spectral diversity. This implies that the system components must be identifiable from their emission spectra. The different imaged components - that is, the different classes in the data images - are not necessarily spatially separated: more than one component may exist in a single resolution cell. This leads to generalized notions of 'segmentation' and 'classification', where the aim is not to partition an image such that each region is assigned to a single class but, for example, to assign to each class a percentage occupation in each resolution cell. This problem may be further complicated when the spectral features of the individual classes are not known a priori, leading to the need for blind processing. Similar problems can arise with other 'multichannel' data sets that involve multiple points of view, time delays, polarizations etc, rather than frequency diversity. This leads to a generalized notion of 'channel', including any possible kind of diversity exploited to form a multidimensional data set. This is an important task for multimedia techniques and as such, is a central topic for MUSCLE.
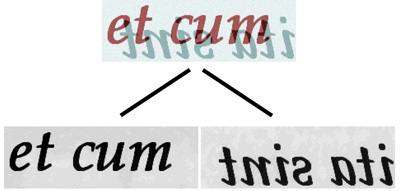
The scope of this kind of analysis is being widened continuously, well beyond the original concerns of multispectral and hyperspectral data analysis. Among the applications for which this type of approach has recently been proposed are astrophysical image processing, remote-sensed image analysis, digital analysis of documents and artworks, medical and industrial diagnostics and computational biology. Several approaches have been used in attempts to solve these problems, often relying on statistics to overcome the lack of specific information. Among the solution techniques we find component and factor analysis, generalized Kalman filtering, particle filtering, Monte Carlo Markov chains and other Bayesian approaches. The activity of the MUSCLE e-team on unsupervised segmentation and classification deals with all these topics, with particular reference to Monte Carlo techniques and astrophysical imagery. The participants are from the 'Alessandro Faedo' Institute of Information Science and Technologies of CNR in Pisa, Italy, and from Trinity College, Dublin. The aim of the CLeMUS session was to encourage the cross-fertilization of ideas coming from the different application areas.
All these considerations have been confirmed in light of the contributions that were received. There were five presentations from the fields of remote sensing, astrophysical imaging, blind source separation and deconvolution, and medical image processing. Very diverse approaches were adopted, such as Bayesian MAP estimation, the use of statistical measures on the observed data, mathematical morphology and multiresolution classification. However, within this variety of methods several commonalities can be observed, which encourages us to pursue further initiatives similar to CLeMUS in the hope that adopting a common viewpoint to study these problems will help us to develop new and unified theoretical and technical tools to solve them. Apart from the common use of diversity data, we find that a popular attitude towards unsupervised tasks, especially when no known data model is assumed, is to rely on statistics and, in particular, on statistical features such as independence, decorrelation or Gaussianity. Two contributions made specific reference to the spatial structure of the class images, through either a Markov random field model or a nontrivial spatial autocorrelation. The extended notion of classification mentioned above was adopted in three contributions, while the other two assumed spatially separated segments. In studying the details more closely, many other common points would be found. Interested readers will find the papers published in Volume 4694 of the Springer Lecture Notes in Artificial Intelligence series.
Links:
http://www.muscle-noe.org
http://kes2007.kesinternational.org
Please contact:
Emanuele Salerno
ISTI-CNR, Italy
E-mail: emanuele.salernoisti.cnr.it
Simon Wilson
Trinity College Dublin, Ireland
E-mail: swilsontcd.ie