by Olga Vybornova, Monica Gemo and Benoit Macq
A new method of multi-modal, multi-level fusion that integrates contextual information obtained from spoken input and visual scene analysis is being developed at Université Catholique de Louvain in the frame of the EU-funded SIMILAR Network of Excellence. An example for application is the "Intelligent diary", which will assist elderly people living alone to perform their daily activities, prolong their safety, security and personal autonomy, and support social cohesion
A system whose objective is to interact naturally with humans must be able to interpret human behaviour. This means extracting information arriving simultaneously from different communication modalities and combining them into one or more unified and coherent representations of the user's intention. Our context-aware user-centered application should accept spontaneous multi-modal input speech, 3D gestures (pointing, iconic, possibly metaphoric) and physical action; it should react to events, identify the user's preferences, recognize his/her intentions, possibly predict the user's behaviour and generate the system's own response. In our case, namely the intelligent diary, we have a restricted domain of application, but must deal with unrestricted natural human behaviour - spontaneous spoken input and gesture.
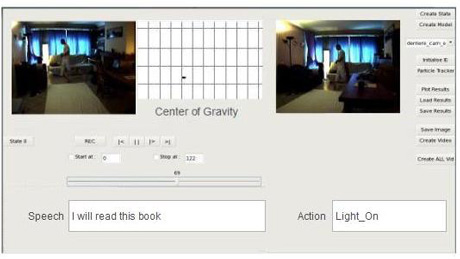
Multimodal fusion is a central question that must be solved in order to provide users with more advanced and natural interaction support. To understand and formalize the coordination and cooperation between modalities involved in the same multi-modal interface (ie an interface using at least two different modalities for input and/or output), it is necessary to extract relevant features from signal representations and thereafter to proceed to high-level or "semantic" fusion. High-level fusion of modalities involves merging semantic content obtained from multiple streams to build a joint interpretation of the multi-modal behaviour of users.
Signal-level and semantic-level processing are deemed to be tightly interrelated, since signals are seen as bearers of meaning. In practical applications it is convenient to distinguish between the two levels of abstraction. In many situations there is a tradeoff, and higher efficiency can be achieved with a better balance between low-level (signal) fusion and high-level (semantic) fusion. To tackle this problem we are complementing our initial approach on optimal feature selection, developed in the information theory framework for multi-modal signals, with a knowledge-based approach to high-level fusion.
The SIMILAR network of excellence provides algorithms that integrate common meaning representations derived from speech, gesture and other modalities into a combined final interpretation. The higher-level fusion operation requires a framework of common meaning representations for all modalities, and a well-defined operation to combine partial meanings arriving from different signals. In our case, the resulting fused semantic representation should contain consistent information about the user's activity, speech, localization, physical and emotional state, and so on.
Everything said or done is meaningful only in its particular context. To accomplish the task of semantic fusion we are taking into account information obtained from at least three contexts. The first is domain context: personalized prior knowledge of the domain such as predefined action patterns, adaptive user profiles, situation modelling, and a priori developed and dynamically updated ontologies for a particular person that define subjects, objects, activities and relations between them. The second is linguistic context, which is derived from the semantic analysis of natural language. Finally, visual context is important: capturing the user's gesture or action in the observation scene as well as eye-gaze tracking to identify salient objects of the activity.
To derive contextual information from spoken input we extract natural language semantic representations and map them onto the restricted domain ontology. This information is then processed together with visual scene input for multimodal reference resolution. The ontology allows the sharing of contextual information within the domain and serves as a meta-model for Bayesian networks that are used to analyse and combine the modalities of interest. With the help of probabilistic weighting of multi-modal data streams we obtain robust contextual fusion. We are thus able to recognize the user's intentions, to predict behaviour, to provide reliable interpretations and to reason about the cognitive status of the person. Figure 1 illustrates an example of multi-modal semantic integration of visual and speech input streams. The system interprets the user's behaviour (action) after analysing contextual information about the user's location (visual context) and intended goal, as derived from the spoken utterance.
Based on our experimental tests, we suggest that in order to make the multi-modal semantic integration more efficient and practical, special attention should be paid to the stages preceding the final fusion stage. The strategy here is to use visual context simultaneously with speech recognition. This helps to make speech recognition more accurate and thereby obtain a text of proper quality.
Our current research is devoted to the implementation of multi-level cross-modal fusion, which looks promising from the point of view of resolving reference ambiguity before the final fusion. It is cross-modal fusion that aids in speech recognition for the elderly, where problems are caused by age-related decline of language production ability (eg difficulties in retrieving appropriate (familiar) words, or tip-of-the-tongue states when a person produces one or more incorrect sounds in a word). This is possible because information from other modalities refines the language analysis at early stages of recognition. Methods for achieving robust and effective semantic integration between the linguistic and visual context of the interaction will be further explored.
Link:
http://www.similar.cc
Please contact:
Olga Vybornova, Universite Catholique de Louvain, Belgium
Tel: +32 10 47 81 23
E-mail: vybornovatele.ucl.ac.be
Link:
http://www.sapir.eu/
Please contact:
Claudio Gennaro
ISTI-CNR, Italy
E-mail: claudio.gennaroisti.cnr.it