The MUSCLE Network of Excellence (Multimedia Understanding through Semantics, Computation and Learning), coordinated by INRIA and ERCIM, presented its activities and showcases on a booth at CeBIT, the largest IT fair worldwide, from 15-21 March 2007 in Hannover, Germany. As part of its dissemination activities, various partners of the network presented their multimedia expertise through interactive demonstrations.
Live demos allowed visitors to try out and experiment with 'Maps of Music' exploring musical landscapes and listening to selected music using the PlaySOM application and the 'PocketSOMPlayer' developed at Technical University Vienna. By applying self-organising maps (SOM) - a subtype of artificial neural networks - to automatically analyzed audio content (frequency spectra), researchers have created an innovative way to organize music by sound similarity. After categorization, the content of an audio collection is displayed on the screen of a laptop or mobile device as a topographic map where colour represents the density of a particular genre in the collection. The user can then select a playlist reflecting his or her mood by drawing a path through this audio landscape.
A research group at the EC3 - E-Commerce Competence Center, Vienna, Austria, demonstrated 'The Media Square', a SOM-based 3D Virtual World allowing users from all over the world listening to music together as well as attending meetings in a virtual world.
INRIA presented a video-clip copy detection software that identifies copied parts in a video even if it was remastered. This is a useful tool to control the copyrights of digital contents on the web and on TV, and also a powerful tool to analyze and explore these data. A crucial difficulty is the fundamental difference between a copy and the notion of similar image encountered in Content-Based Retrieval: a copy is not an identical or a near replicated video sequence but rather a transformed video sequence. These photometric or geometric transformations (gamma and contrast transformations, overlay, shift, etc) can greatly modify the signal, and therefore a copy can in fact be visually less similar than other kinds of videos that might be considered similar.
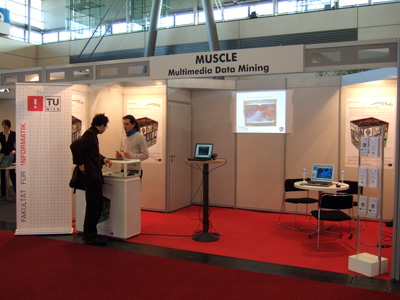
MUSCLE aims at establishing and fostering closer collaboration between research groups in multimedia data mining and machine learning. The goal is to explore the full potential of statistical learning and cross-modal interaction for the (semi)-automatic generation of robust meta-data with high semantic value for multimedia documents.
Link:
http://www.muscle-noe.org/
Please contact:
Patricia Ho-Hune, MUSCLE project manager
ERCIM office
E-mail: patricia.ho-huneercim.org