by Rob van der Mei and Thije van Barneveld
Research into the fields of information and communications technology and applied mathematics is very relevant for today’s society. At CWI, we have pursued investigations in these fields to enhance the vital services provided by ambulances through the development of Dynamic Ambulance Management.
In life-threatening emergency situations, the ability of ambulance service providers (ASPs) to arrive at an emergency scene within a short period of time can make the difference between survival or death for the patient(s). In line with this, a service-level target is commonly used that states that for high-emergency calls, the response time, i.e., the time between an emergency call being placed and an ambulance arriving at the scene, should be less than 15 minutes in 95% of cases. To realise such short response times, but still ensure running costs remain affordable, it is critical to efficiently plan ambulance services. This encompasses a variety of planning problems at the tactical, strategic and operational levels. Typical questions that must be answered include “How can we reliably predict emergency call volumes over time and space?”, “How can we properly anticipate and respond to peaks in call volumes?”, “How many ambulances are needed and where should they be stationed?”, and “How should ambulance vehicles and personnel be effectively scheduled?”.
A factor that further complicates this type of planning problems is uncertainty, an ever-present consideration that in this context, will affect the entire ambulance service-provisioning process (e.g., emergency call-arrival patterns, travel times, etc.). The issue is that the planning methods currently available typically assume that “demand” (in the context of ambulance services this would refer to call volumes and their geographical spread) and “supply” (the availability of vehicles and ambulance personnel at the right time in the right place) parameters are known a priori. This make these methods highly vulnerable to randomness or uncertainty, and the impacts this inevitably has on the broader planning process, namely inefficiencies, and higher costs. For ambulance services, the challenge is to develop new planning methods that are both scalable and robust against the inherent randomness associated with the service process, both real and non-real time.
A highly promising development that is gaining momentum in the ambulance sector is the emergence of Dynamic Ambulance Management (DAM). The basic idea of DAM is that ambulance vehicles are proactively relocated to achieve a good spatial coverage of services in real time. By using dynamic and proactive relocation strategies, shorter arrival times can be achieved [1].
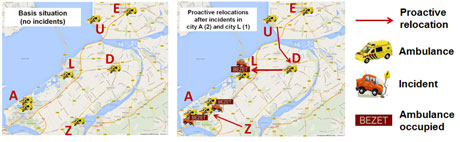
Figure 1: Illustration of proactive relocations of ambulance vehicles.
To illustrate the use of DAM, consider the following example area which features six cities (A, D, E, L, U and Z; Figure 1), serviced by seven ambulances. When there are no emergencies (a ‘standard situation’), optimal vehicle coverage is obtained by positioning one ambulance in each of the six cities, with one additional vehicle in city A as it has the largest population. Now consider a scenario where an incident occurs at city L while simultaneously, two additional incidents are occurring in city A. These incidents can all be serviced by the ambulances currently located in those two cities. Under an ‘optimal’ dynamic relocation policy, this scenario should then trigger a proactive move by the ambulance in city D to city L, in order to maintain service coverage. As soon as that ambulance is within a 15-minute driving range of city L, the ambulance at city U should move to city D (note that city U is smaller than D, meaning that it can be covered by city E’s ambulance). The ambulance at city Z can then proactively move to city A. This example illustrates the complexity in using DAM: for example, what additional steps would be appropriate if an additional accident was to occur in city U whilst the ambulance was transitioning between cities U and D?
The key challenge in an approach such as DAM is developing efficient algorithms that support real-time decision making. The fundamental question is “under what circumstances should proactive relocations be performed, and how effective are these relocation actions?”. Using methods from the stochastic optimization techniques Markov Decision Processes and Approximate Dynamic Programming, we have developed new heuristics for DAM [2,3]. Implementation of these solutions in the visualization and simulation package suggests that strong improvements in service quality can be realised, when compared with the outcomes gained from the relocation rules currently being deployed by most ambulance providers. Currently we are in the field trial phase and are setting up a number of newly developed DAM algorithms, in collaboration with a number of ASPs in the Netherlands. These activities are part of the project ‘From Reactive to Proactive Planning of Ambulance Services’, partly funded by the Dutch agency Stichting Technologie & Wetenschap.
Links:
http://repro.project.cwi.nl
Dutch movie: http://www.wetenschap24.nl/programmas/de-kennis-van-nu-tv/onderwerpen/2014/april/wiskunde-redt-mensenlevens.html
References:
[1] M. S. Maxwell et al.: “Approximate dynamic programming for ambulance redeployment”, INFORMS Journal on Computing 22 (2) (2010) 266-281
[2] C.J. Jagtenberg, S. Bhulai, R.D. van der Mei: “A polynomial-time method for real-time ambulance redeployment”, submitted.
[3] T.C. van Barneveld, S. Bhulai, R.D. van der Mei: “A heuristic method for minimizing average response times in dynamic ambulance management”, submitted.
Please contact:
Rob van der Mei
CWI, The Netherlands
E-mail: