by Paolo Santi, Giovanni Resta and Carlo Ratti
Shareability networks demonstrate that more than 95% of taxi trips taken in New York can be shared with minimal passenger discomfort.
The increasing pervasiveness of digitalized information has unleashed unprecedented opportunities for understanding aspects of human behaviours and social lives, including individual mobility. An enormous amount of digital traces are now obtainable from a range of sources (e.g., cell phone records, taxi GPS traces, etc.) which allows human mobility to be analyzed to an extent that would have been inconceivable several years ago [1]. Although this raises privacy concerns, this “big data” era offers unique opportunities to improve understanding around human mobility needs and, hence, improve transportation system efficiencies.
The goal of this project, performed in cooperation with a team from the MIT Senseable City Lab (Michael Szell, Stan Sobolevsky and coordinated by Carlo Ratti), is to quantify the benefits of taxi sharing in New York City (NYC). Our analysis was based on a dataset which captured all the taxi trips taken in NYC in 2011 (over 150 million trips). For each trip, the dataset captures the pick-up time and location and drop-off time and location.
In this project, we posed the fundamental question, “How many taxi trips can be shared in NYC?”. To answer this question, the intrinsic trade-off between shareability opportunities and passenger discomfort must be considered: the longer a passenger is willing to wait for a shared trip, the higher the sharing opportunities. This tradeoff is made explicit by the novel notion of a shareability network in which we have defined the model sharing opportunities: each network node represent a separate trip and links between two nodes represents a sharing opportunity between those trips. The criterion used to determine whether two trips can be shared is based on spatial and temporal constraints. For two trips, T1 and T2, a sharing opportunity only exists if a route connects the respective pick-up and drop-off points such that both passenger groups can be picked up and delivered to their destinations with a delay of no more than ,where explicitly models the tradeoff between passenger discomfort and shareability. A higher value of results in a denser shareability network and corresponds to more opportunities for trip sharing.
Using a shareability network allows an optimal solution to be found to an otherwise computationally intractable problem. Shareability networks impose a structure to an otherwise unstructured, immense search space by constraining the number of trips that can be shared (up to k, where k is a user-defined parameter, set to 2 or 3 in this study) and considering only static trip sharing. This term means that once two or more trips are combined into a shared trip, the combined trip is served by a single taxi that cannot be rerouted for further sharing. Once the search space has been reduced and structured, an optimal trip sharing figure can be computed in approximately 0.1 seconds by running a computationally efficient maximum matching algorithm on the shareability network (10,000 nodes and 100,000 links) [2] using a standard Linux workstation. Thus, our proposed methodology is suitable for real-time implementation.
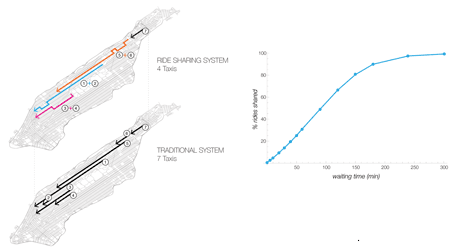
A notable result of our analysis is that constraining the search space to reduce computational complexity does not impair trip sharing opportunities. The percentage of shareable trips is shown to increase with the delay parameter and we found it was as high as 95% if a delay in the order of five minutes was permitted. Thus, the vast majority of taxi trips taken in NYC can be shared with minimal passenger discomfort.
We also investigated the effects of the sharing penetration rate which accounts for the true fraction of passengers that want to use a shared taxi service. We are extending this analysis to other cities (Singapore, Vienna, San Francisco, etc.) to investigate whether similar sharing opportunities arise in other urban contexts.
References:
[1] M. Gonzales, C. Hidalgo, A.L. Barabasi, “Understanding Individual Human Mobility Patterns”, Nature, 2008
[2] Z. Galil, “Efficient Algorithms for Finding Maximum Matching in Graphs”, ACM Comp. Surv. 1986.
Please contact:
Paolo Santi
Istituto di Informatica e Telematica and MIT Senseable City Lab
E-mail: