by Marcell Fehér and Bertalan Forstner
Worldwide, the one billion cars in use emit enormous amounts of carbon dioxide. Potentially these emissions could be drastically reduced if people shared their daily commutes. The idea of carpooling has been around for a long time and already, millions of gallons of gas have been saved, but the widespread adoption of this strategy is yet to come. Here, we introduce a carpooling service which is easy to use and aims to minimize user effort, thus encouraging the switch to this smart, environmentally friendly transportation option.
In recent history, cars have become our primary choice of transport. However, this high number of motor vehicles have become a significant contributor to climate change, emitting approximately five billion tons of carbon every year. The transportation sector currently accounts for approximately 15% of overall greenhouse gas emissions, a figure which grew by 45% between 1999 and 2007. While getting drivers to swap the comforts of a car for public transport seems like an unattainable goal, encouraging them to carpool is a more appealing prospect and with an estimated 3.75 empty seats per car, per trip [1], the efficiency potential is significant. Changing the mindset of car commuters might not be the task of computer science, but making carpooling services more accessible definitely is. In the era of smartphones, with location tracking abilities and always-on connectivity, providing the public with an easy-to-use recommendation system is no longer impossible.
According to our vision here at the Budapest University of Technology and Economics, the ideal computer-aided carpooling system should require very little effort from the users. In the last two years, research engineers at the Department of Automation and Applied Informatics developed the underlying algorithms such a system would use, and we describe that system below.
It depends on a light-weight application runs constantly on the smartphones of both the drivers and the passengers, tracking their locations and learning their transport usage habits. This data is not uploaded to any server, but rather movement patterns are extracted on the phone itself using advanced, mobile-optimized algorithms. The recurring patterns, identified as routines, consist of a day reference (i.e., what days of the week they happen on), the approximate time window (e.g., between 7:30 and 8:20) and the actual route typically taken by the user.
When a user wants to either volunteer as a driver or seek a ride, they can securely upload their routines to a central server, where the matching process takes place. Here, the routines of the drivers and passengers are compared to find good matches which are then suggested to the user. For example, the usual departure and destination locations of Passenger X are indicated by blue markers in Figure 1. After cross-comparing his departure and destination preferences with the morning routines of drivers in the same area, the system found two potential cars Passenger X may be able to join (displayed by the red and green tracks). Note that the full routes are intentionally not shown since they considered sensitive, private information. When Passenger X receives his suggested options, he can clearly see the proposed pick-up and drop-off locations, as well as approximate timings. He can also call the driver using the phone icon displayed next to their name.
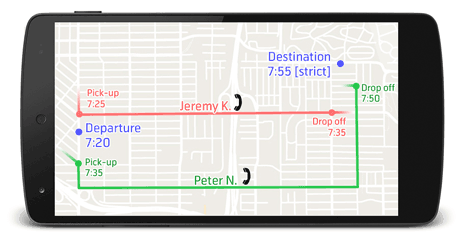
This system assumes that arrival times are strictly held on morning routes since people are typically going to work at this time. Conversely, with afternoon routes, departure times are more important since users don’t want to wait long for their rides after work. Therefore, when a carpooling search is performed for the morning, the system considers the distance between the drop-off and destination points and (using a five kilometer per hour walking speed) calculates the possibility of being able to walk to the destination and arrive before the strict arrival time.
While these carpooling recommendations a very useful feature by themselves, the large set of learned routines enable a much broader family of services. For example, in case of a traffic jam or road works, the system is able to identify their location(s) by identifying the lower car speeds of drivers who usually take that route. Other regular route users could then be automatically notified of such a disruption, thus enabling them to leave at a different time or take a different route. Our team is currently working on supporting several usages of this geo-social information.
Links:
http://amorg.aut.bme.hu/
http://www.internationaltransportforum.org/2010/
Reference:
[1] Mayinger, F. (Ed.). Mobility and Traffic in the 21st Century. Springer.
Please contact:
Marcell Feher
Budapest University of Technology and Economics, Hungary
E-mail: