by Anders Holst
A new model to detect and visualize ships behaving in a strange or suspicious manner can help authorities to prevent or mitigate accidents and detect illegal activities at sea.
The three-year SADV project, which finished in 2013, has been a collaboration between SICS Swedish ICT, Saab AB, the Swedish Coast Guard, the Swedish Customs Service, the Swedish Armed Forces and the Swedish Space Corporation. The aim of the project has been to support the maritime surveillance operators by providing them with an increased situation awareness. This is done using automatic tools which detect vessels bahaving suspiciously or uncharacteristically. Increasing volumes of maritime traffic, combined with threats from criminal activities and environmental issues, just to name a few, there is a high demand for tools that can support the real-time analysis of the huge amounts of maritime situation data that are produced.
Three approaches for anomaly detection
There are essentially three different approaches to detect anomalies and the framework developed in this study supports all three:
1. statistical anomaly detection, where a statistical model is built on normal situations and situations that are very unlikely to come from that model are considered anomalies;
2. rule based anomaly detection, where rules are designed to detect situations of interest; and
3. model based (or simulator based) anomaly detection, where real observations are compared to simulated results (i.e., what would have happened in a normal situation). A difference indicates an anomaly.
While it is easily seen that approaches two and three require extensive expert involvement to design the rules and simulator, approach one would appear to require no expert involvement. However, this approach still requires extensive domain knowledge, first to determine what kind of anomalies would be interesting to detect and secondly, to ensure that the model considers the features that are relevant for detecting those anomalies. Typically, the data requires some processing as these features are not directly represented in the raw data.
Uncharacteristic behaviours considered
A number of uncharacteristic vessel behaviours have been considered in this study. They are:
- unusual speeds and directions (e.g., going the wrong direction in a route),
- unusual movement patterns (e.g., too many stops or turns at-sea),
- making a rendezvous at sea.
- unusual route choices (as compared with other ships), and
- data inconsistencies (e.g., a ship changing identity or using the same identity as another ship).
Each of these behaviours requires different anomaly detectors which consider different features calculated from different parts of the data, often on different time scales. Some are suitably handled with statistical methods, others with rule based approaches and yet others with a combination. Most statistical anomaly detection approaches used in this study were based on the Incremental Stream Clustering framework [1] developed by SICS, and most of the rule based detection is based in the Situation Detector platform [2] developed by Saab.
Results
The methods developed in this study were evaluated on real maritime data collected from the Baltic sea. The success of this approach in detecting interesting situations is illustrated by the following examples:
- A risk of running ashore - Saab has combined a rule based detection of ships approaching shallow waters with a statistical anomaly detector for ships that deviate from the usual routes in the area. The detector detected actual groundings up to three minutes prior to such an event occurring (see Figure 1).
- Dangerous behaviour - the SICS’s movement pattern behaviour analysis detected a small fishing boat that was repeatedly stopping in the middle of a shipping route and moving irregularly in the wrong direction (apparently fishing). It is almost hit by a large freight ship that managed to veer at the last minute (Figure 2).
- Several ships using the same identity - the SICS’s inconsistency detector identified several ships using the wrong identity number. These are mostly genuine mistakes; however, there are examples of ships in other parts of the world intentionally changing their identity numbers to circumvent trading embargoes.
- Meetings between ships at sea - most meetings, unless they are between certain ship types (i.e., coast guard, rescue vessels, etc.), may potentially indicate illegal activities such as smuggling or possibly trawling in prohibited areas.
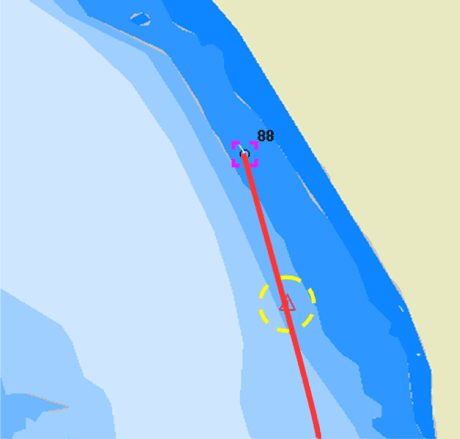
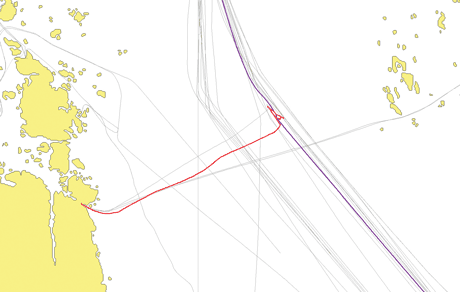
How the model works
The task of combining all three different types of anomaly detection in one framework was not trivial, but we have managed to design a general architecture that fits with several existing maritime surveillance platforms and is also able to handle several anomaly detectors of different kinds. The architecture of the anomaly detection module is schematically shown in Figure 3.
The key to this architecture is identifying information flows that are common across all the surveillance platforms and for all kinds of anomaly detectors. All detectors need situation data from the platform, and they need to provide anomaly indications back, based on that data. They also need to support user configuration and inspection.
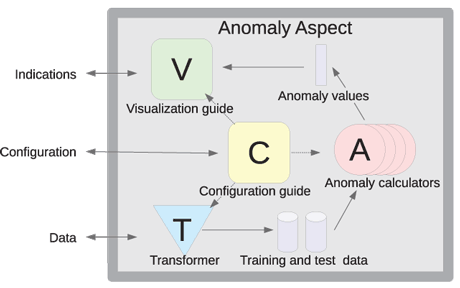
The next step is to identify similarities between the different kinds of anomaly detectors. They all need to extract the relevant features from the provided data, use their respective methods to determine whether those features represent an anomaly, and then finally transform that judgement into an “indication” which is complete with details of what, when and why.
If the indications from one anomaly detector can be used as an input feature to another anomaly detector, it is possible to combine the rule based and statistical detectors in a more advanced ways, as opposed to just having them run in parallel in the same system [3]; statistical detections can be referred to in a rule, or the number of times that a rule matches can be considered in a statistical detector.
Conclusion
The SADV project has significantly extended previous methods used for anomaly detection within the maritime surveillance sphere and shown that these methods can detect interesting and relevant situations.
Link:
http://www.sics.se/projects/sadv-statistical-anomaly-detection-and-visualization-for-maritime-domain-awareness
References:
[1] A. Holst and J. Ekman: “Incremental stream clustering for anomaly detection and classification”, in Eleventh Scandinavian Conference on Artificial Intelligence, SCAI 2011
[2] J. Edlund, M. Grönkvist, A. Lingvall and E. Sviestins: “Rule-based situation assessment for sea surveillance”, in proc. of SPIE Defence and Security Symposium 2006
[3] A. Holst et al.: “A Joint Statistical and Symbolic Anomaly Detection System: Increasing performance in maritime surveillance”, in FUSION 2012.
Please contact:
Anders Holst
SICS Swedish ICT
E-mail: