by Daniele Fontanelli, Luca Greco and Luigi Palopoli
Two trends can be recognized in the recent development of embedded control systems: the adoption of sophisticated sensing systems (which require large and highly variable processing times) and the proliferation of control applications that are deployed on the system. The combination of the two trends has caused an obsolescence of hard real-time design techniques, which allocate computing resources based on the worst case demand of the application. There exists an effective replacement, however, that allows us to reconcile performance guarantees and efficiency in resource management.
An ever growing number of control applications utilize sophisticated sensing devices that can extract multiple information from each measurement. For instance, visual sensors are in charge of device localization, people detection and the extraction of features of interest for control purposes (e.g., the lines delimiting a lane in an automated driving system).
The price paid for the adoption of such complex devices is a large and highly variable processing time. Indeed, in a “clean” environment, the amount of computation required to extract the relevant features can be very low, while it skyrockets in the presence of a large number of artifacts or under dim illumination.
The need for sharing
Equally important is a different requirement of modern control systems: to maximize hardware sharing between the different control functions. In a modern car, for instance, the complexity of the communication infrastructure is currently measurable in kilometres of copper cables and hundreds of kilograms of weight. The same applies to Electronic Control Units (ECU) used to execute computations, whose number easily exceeds 100.
Positioning and interconnecting of these devices has a tremendous impact on the complexity of system engineering. Hardware complexity has reached the critical point where it becomes imperative for new or more advanced control functions to exploit the existing computation and communication infrastructure.
Limits of classic approaches
With strong fluctuations in the computing workload on one side, and the need for intense hardware sharing on the other, the designer is confronted with problems that can hardly be addressed by traditional design approaches. The classic mandate is to grant to each control function hardware resources commensurate to its worst case demand (e.g., a dark and cluttered scene for visual sensors). This way, it will be executed with regular timing and with constant delays, thus allowing control designers to formulate and enforce guarantees on control performance. The rigid application of this old paradigm to the new context naturally leads to a massive waste of resources (which lie unused for most of the time) and to a drastic reduction in hardware sharing opportunities. On the contrary, if the control function receives resources proportionate only to its average requirements, there can potentially be large intervals of time when the system is not properly controlled: performance and even stability could be lost!
A stochastic approach
The University of Trento and the University of Paris Sud, within the framework of the FP7 HYCON2 project (running from 2010 to 2014), has started a research activity which aims at reconciling performance guarantees and hardware sharing. The key idea underlying this research is that the limits of classic methods can be overcome by a suitable stochastic description of the computation requirements. Clearly the new approach calls for new performance metrics (e.g. Almost-Sure Stability and Second Moment Stability), which are themselves stochastic in nature. Broadly speaking, system trajectories are allowed to have stochastic fluctuations, but eventually they will behave properly (except for a small and statistically irrelevant set of them). The application of these notions to our context needed a formal mathematical model to describe the stochastic evolution of the delays incurred in control computation. In [1,2] we met this requirement by combining a soft real-time scheduler [3] with an appropriate programming discipline. The former guarantees that the different computing tasks will have timely access to the computation resources, while the latter forces interactions between computers and environment to take place on well defined instants. Leveraging these ideas, it was possible to establish a connection between the stochastic control properties and the fraction of computing resources to allocate.
A concrete case study
We considered a mobile robot (Figure 1) that was required to follow a black line drawn on the ground. The variability in the scene background determines a similar variability in the computation time (Figure 2). In the worst case, the computation time is beyond 40ms; if the system is operated at 25 frames per second this corresponds to a worst-case utilization beyond 100%. With the classic approach, the control function would require the allocation of the entire CPU. Our methodology has revealed that 21% of CPU utilization is in fact sufficient to stabilize the system.
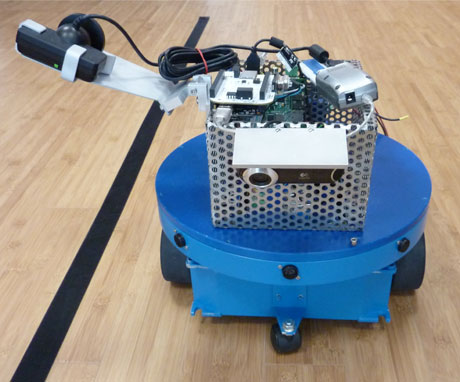
Figure 1: The actual robot adopted for the case study.
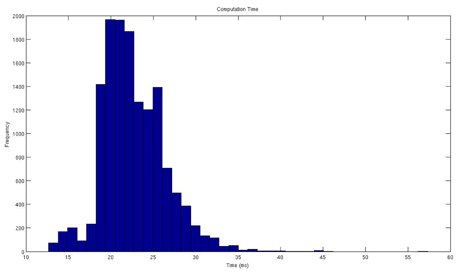
Figure 2: Probability mass function of the computation times observed during the experiments
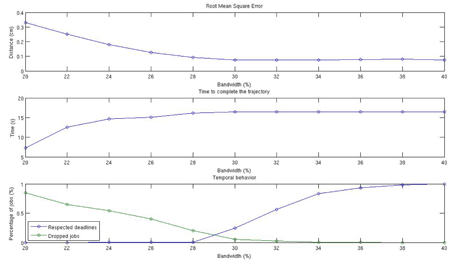
Figure 3: Experimental results achieved with different bandwidth values: root mean square of the deviation from the desired position (top); time the robot succeeds to remain on the track (middle); percentage of task executions that complete within a deadline or that are cancelled (bottom).
In Figure 3 we show the experimental results achieved with different bandwidth values. The top plot reports the integral of the root mean square of the deviation from the desired position, while the middle plot reports the time the robot succeeds to remain on the track (the duration of the experiment is 40s). The performance dramatically improves when we allocate more than the minimal 21%, but there is no apparent advantage in exceeding 30%. In the bottom plot, we report the percentage of task executions that complete within a deadline equal to the period and the percentage of executions cancelled because of time-out. In the classic setting these figures would be 100% and 0% respectively. In contrast, the plot reveals that the system can operate with provable performance far away from this regime with substantial resource savings.
Conclusion
The experiments here presented show that the stochastic scheduling approach is a viable solution for real-time control of systems, in which the desired control performance can be achieved even with limited computing resources.
References:
[1] D. Fontanelli, L. Greco, L. Palopoli: “Soft RealTime Scheduling for Embedded Control Systems”, Automatica, 49:2330-2338, July 2013.
[2] D. Fontanelli, L. Palopoli, L. Greco: “Deterministic and Stochastic QoS Provision for Real-Time Control Systems”, in proc. or RTAS 20111, Chicago, IL, USA, p. 103-112
[3] R. Rajkumar et al.: “Resource kernels: A resource-centric approach to real-time and multimedia systems”, in proc. of the SPIE/ACM Conference on Multimedia Computing and Networking, 1998.
Please contact:
Daniele Fontanelli, Luigi Palopoli, Università di Trento, Italy
E-mail:
Luca Greco, L2S Supélec, Paris, France
E-mail: