by Marco Pellegrini
Imagine that searching for videos by specifying visual content is as easy as searching for Web pages by specifying keywords (as is done with search engines like Google and Yahoo). This is the Holy Grail of video searching by content. In the Internet of the Future (IOF), architectural support for multimedia will be influenced by new user-centric search paradigms. The VISTO project is a step towards this goal.
Video browsing and searching is rapidly becoming a very popular activity on the Web. This has led to the need for a concise searchable, browsable and indexable video content representation, as well as new IOF architectures to support it.
YouTube, the most famous Web-based repository of (short) videos, is growing at breakneck pace. In March 2008, the total number of videos uploaded was 78.3 million, with over 150,000 new videos being uploaded every day. On average each video runs for 2 minutes 46 seconds, meaning it would take about 412 years to view all of the material uploaded on YouTube. Clearly any practical system devoted to indexing video data sets of YouTube's size must function on a time scale orders of magnitude smaller than the duration of the video being processed.
New paradigms of user behaviour induced by new searching tools will have a strong impact on the future of the Internet. Video content is already one of the largest contributors to Internet traffic and several architectural solutions are being proposed to handle it (centralized server-based, grid-based, cloud computing, peer-to-peer etc). Indexing, searching-by-content and delivery of multimedia data represent a key application driving architectural trade-off choices for the IOF.
Managing large video repositories involves finding solutions for several aspects:
- compression, coding, transmission
- synthesis and recognition
- storage and retrieval
- access (interface, matching user and machine)
- searching (based on machine intelligence)
- browsing (based on human intelligence).
The Visto project is focused on introducing innovative solutions for video indexing to improve the speed and accuracy of searching and browsing. VISTO is intended as a distributed application to be optimized on IOF architecture.
Currently for browsing purposes, static video summarization techniques can be used. Unfortunately, state-of-the-art techniques require significant processing time, meaning all such summaries are currently produced in a centralized manner, off-line and in advance, without any user customization. With an increasing number of videos in video collections and with a large population of heterogeneous users, this is an obvious limit. In the VISTO project, we employ summarization techniques that produce high-quality customized, indexable and searchable on-the-fly video storyboards. The basic mechanism uses innovative fast clustering algorithms that select, on the fly, the most representative frames using adaptive feature vector spaces. Such techniques are naturally embeddable into distributed scenarios.
Browsing activity: browsing involves an aspect of serendipity in which the user needs to quickly sift through large numbers of videos (say ten or twenty) in order to detect those of interest. Summaries must be made quickly (on the fly) and be of high quality. Users can select basic parameters such as the storyboard length and processing time, as well as advanced ones such as the similarity metric, the dynamic (motion-flow) content and other features. Moreover, use of personalized criteria is made possible by the fact that very little pre-computation is needed. Preliminary objective and subjective evaluations of basic principles show that storyboards produced on the fly can be of high quality.
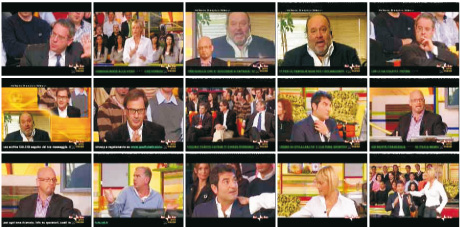
Searching activity: the user may encounter a frame/scene during video-browsing that is of particular interest (eg a city skyline) and be curious as to whether other videos contain a similar scene/frame (eg a different take of the same city skyline). At this point the user could extract new and unexpected knowledge from a comparison of different videos having this key common scene/frame.
Personalization of browsing and searching: given a video that is of interest for a user, browsing involves being able to quickly assess the most interesting (relevant, typical or unusual) frames/scenes in the video in order to decide whether it is worth watching in its entirety (or which portions are worth viewing). Users should be allowed to select at view-time basic parameters such as storyboard length and waiting time. However more advanced summarization criteria need to be supported. For example users must be allowed to specify the dynamic (motion-flow) content they are interested in (eg a moving car is different from a parked one). Further, the underlying similarity metric used for selecting the representative frames should be biased using implicit or explicit user requirements.
Videos can be considered to be sequences of still images (with sound), and techniques for handling large collections of still images (pictures) might be the first line of attack. However, we do not consider this approach to be suitable. A single video corresponds to thousands/millions of frames (depending on its duration) that, with the exception of scene changes, are locally highly similar to one another. Thus the sheer volume of data poses scalability problems to approaches based on indexing single frames (or a dense blind sample of them) as single still images. Instead, in the VISTO project, we intend to develop video representations and indexing techniques that take dynamic components of the video data as prominent in the representation. The aim is to simultaneously exploit the spatial and temporal coherence of video data, not only to attain compression, but also to make searching fast and efficient. Attaining both goals simultaneously is challenging.
Future activities will involve setting up a complete network-aware software/ hardware/conceptual architecture able to cope with high throughput demands in a P2P style of computation, taking advantage of IOF architectural designs. Awareness of network capabilities, which change over time, is particularly important for use on, for example, wireless hand-held devices.
The VISTO project started in 2007 at IIT-CNR as a collaboration between researchers of the Institute for Informatics and Telematics, CNR, Pisa, the University of Modena and Reggio Emilia, and the University of Piemonte Orientale.
Link:
http://visto.iit.cnr.it/
Please contact:
Marco Pellegrini
IIT CNR, Italy
E-mail: marco.pellegriniiit.cnr.it