Remote sensing is making an increasingly significant contribution to the mapping and monitoring of vegetation. Satellite and aircraft platforms are able to quickly gather very large amounts of data; in addition, remotely sensed data can be archived so that it is possible to track changes in the Earth's surface over long periods of time.
One of the most important advances of the last decade is the availability of multispectral and hyperspectral sensors able to measure the radiance emitted by the Earth's surface in several narrow spectral bands. An important use of remotely sensed image data is the monitoring of land coverage for diverse applications: deforestation, agriculture, fire alerting etc. Other applications include land classification and segmentation, which are useful in fields such as land-use management and the monitoring of urban areas, glacier surfaces and so on. A lot of information can be extracted from these images and applied, for example, in monitoring soil conditions, supporting cultivation management or mapping land usage.
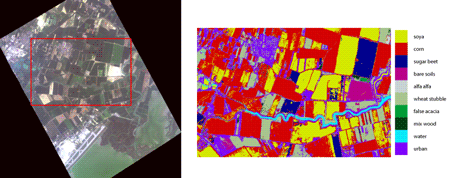
Land classification exploits the fact that we are able to identify the type of land cover associated with a given pixel by its unique spectral signature. The use of medium- or high-resolution spectral data opens new application opportunities: coverage of a wider fraction of the electromagnetic spectrum at a better spectral resolution means a better representation of the spectral signature corresponding to each pixel and better recognition of the unique spectral features of land categories.
However, the effectiveness of standard univariate classification techniques applied to multispectral data is often hindered by redundant or irrelevant information present in the multivariate data set.
Processing a large number of bands can paradoxically result in a higher classification error than processing a subset of relevant bands without redundancy, if multispectrality is not correctly taken into account.
Image processing can also provide boundary recognition and localization. One of the most frequent steps in deriving information from images is segmentation: the image is divided into homogeneous regions that are estimated to correspond to structural units in the scene, and the edges to be detected are calculated to correspond to the contours of these units. In some cases, segmentation can be performed using multiple original images of the same scene (the most familiar example is that of colour imaging). For satellite imaging this may include several infrared bands, containing important information for selecting regions according to vegetation, mineral type and so forth.
The WAGRIT project has been developed within the framework of the Italian Space Agency funding program to support SME technology development. The main functions of WAGRIT are: authenticated access to data and services via Intranet/Internet; multi-platform client applications; raster and GIS data browsing and retrieval from centralized archives; centralized data processing on the server side; data handling and editing on the client side; and simultaneous visualization of up to four images and synchronized operations (pan, zoom etc). In particular, the project has addressed the question of supervised classification based on statistical approaches and of land segmentation based on a variational approach.
Discriminant analysis was used in the first case. It was applied in the context of vegetation detection to establish relationships between ground and spectral classes. We have extended classical discriminant analysis with (a) a linear transform of the original components into principal or independent components, and b) a univariate nonparametric estimate of the density function for each separate component. In this way we are able to circumvent the problem of dimensionality and also to apply discriminant analysis to the land-cover problem where representation of land typology by parametric functions is inadequate.
For the segmentation problem, we chose a variational formulation motivated in part by the desire to combine the processes of edge placement and image smoothing. Indeed, classical edge detection techniques (Marr-Hildreth, Canny and their variants) separated these processes: the image is first smoothed to suppress noise and control the scale, and edges are detected subsequently, for example as gradient maxima. One consequence of this approach is pronounced distortion of the edges, especially at high-curvature locations. Corners tend to retract and to be smoothed out; the connectedness of the edges at T-junctions is lost. By introducing interaction between the edge placement and the smoothing, this effect could be fixed.
Both classification and segmentation methods have been tested on MIVIS (Multispectral Infrared and Visible Imaging Spectrometer) data for which a ground truth validation was available. Figure 1 shows results on an MIVIS hyperspectral image of an area in the neighbourhood of the Venice Airport (image and ground truth data kindly provided by IMAA-CNR). Here a very fine classification, undetectable by the naked eye, is made possible by the large spectral depth of the MIVIS measurements, which is well exploited by the processing scheme.
Please contact:
Maria Francesca Carfora
IAC CNR, Italy
Tel: +39 081 6132389
E-mail: f.carforaiac.cnr.it