The Study Group Mathematics with Industry (SWI) workshops aim at bringing mathematicians (and occasionally computer scientists) together for a week to work on some difficult unsolved industrial problem. Besides reinforcing scientific, industrial and social relations, participants are challenged to come up with a fresh perspective on the problem and more often than not this leads to directly usable results and methods for industry. This year's workshop, organized at the University of Twente in the Netherlands (28 January - 1 February 2008), hosted a project on the real-time classification of single-neuron recordings, jointly proposed by Philips Research and the Amsterdam Academic Medical Centre, who have been collaborating on this topic for some time.
The problem involves helping neurosurgeons get their bearings during deep brain surgery. The method currently used involves inserting fine needles into the brain to record neuron action potentials for periods of about ten seconds, converting the activity to sound waves, and playing it to the surgeon, who then decides whether the needle is on target or not. The two principal aims of the workshop project were to determine which methods of analysis and information presentation would make the life of the surgeon easier by classifying recorded neural activity on the fly; and to incorporate the knowledge of the expert surgeon into the analysis in such a way as to aid inexperienced surgeons. The latter problem is particularly important since expert knowledge is highly qualitative, depends on intuition honed by many surgeries, and is very difficult to encapsulate as a procedural description.
Apart from the modelling of expert knowledge, there are several challenges in this problem. In detecting neural activity a needle records a great deal of background noise, which needs to be accounted for. Deep brain recordings have much higher noise levels than cortical recordings. Depending on the proximity of neurons in the area, several neural activities including cellular action potentials, axonal fibre bundles and field potentials can be recorded with a single needle, and the fact that closely spaced neurons usually have highly correlated activities makes their separation difficult. Even though we can attribute several general characteristics to a neural spike, they have different shapes and firing patterns, which sometimes change with time and almost always overlap with other sources. A single neuron can have relatively regular interspike intervals, or it can be a bursty neuron with alternating periods of low activity and high-frequency firing. Furthermore, neurons can become active or inactive during a single recording, and the number of neurons contributing to the signal may change.
Spike sorting is not a recent problem: it has been around since the 1960s. Early methods relied on template matching, and required heavy offline processing. More recent methods combine feature extraction, probabilistic modelling and clustering. The accuracy and efficiency of these methods are much greater than before, but most are still too computationally intensive to be used during the surgery, and do not work well with deep brain recordings.
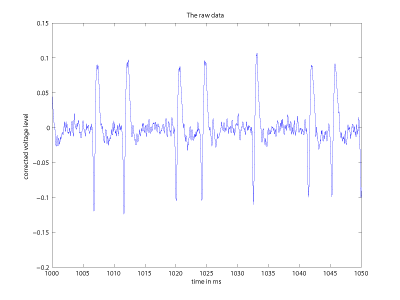
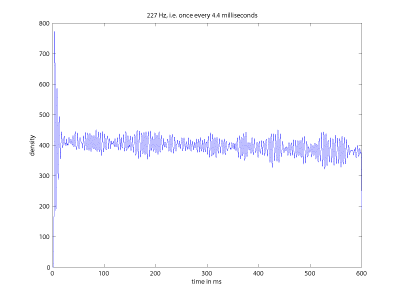
Our study group inspected two main approaches to the problem during the workshop. After the detection and alignment of individual spikes, several features can be extracted from each spike, and used in a classification framework in an unsupervised manner. The spike length, amplitude, total area, polarity, ratio of peaks, or simply the potentials sampled at a fixed rate can serve as features. A Karhunen-Loewe transform followed by a clustering on the projection space produces a fast, clear separation of spike shapes that usually vary smoothly in time. This method is useful even for non-stationary spike shapes, and works well if the shapes are sufficiently discriminative.
Our second approach uses the temporal structure of the spikes. The temporal dependencies can be analysed with Fourier analysis or autocorrelation methods, but the non-stationary nature of the firing rates, irregularities due bursty neurons and the changing number of sources make this task difficult. To robustly identify the trends, we take an approach similar to autocorrelation analysis. We shift the spike sequence repeatedly to align each successive spike with the origin of the temporal axis. We then convolve each spike with a fixed Gaussian function and stack these Gaussians. The peaks of this accumulated function reliably identify periods of neural activity, even if the neuron is inactive from time to time during the recording. Furthermore, the expert surgeon could identify broader trends (eg tremor) in the shift-stack histogram.
We have obtained promising results on a small set of recordings, and even though the workshop is over, the project continues. We are currently working on several extensions, with the hope that some of our ideas may eventually make their way into the operating room.
Links:
http://wwwhome.math.utwente.nl/~swi2008/
http://www.amc.uva.nl
http://www.research.philips.com
http://homepages.cwi.nl/~salah/
Please contact:
Albert Ali Salah
CWI, The Netherlands
Tel: + 31 20 592 4214
E-mail: a.a.salahcwi.nl