by Poulicos Prastacos and Yiannis Kamarianakis
Intelligent transportation systems integrate data collection, processing and communications infrastructure with data storage and analytical tools. At IACM-FORTH, researchers in the Regional Analysis Division (RAD) have been working on the statistical modelling issues that emerge in the processing of traffic data streams of great magnitude and wildly varying quality. In particular, they are looking at the detection of traffic sensor malfunctions, imputation of missing or bad data, estimation of velocity and forecasting of travel times in urban arterials.
As vehicular traffic congestion has increased in recent decades, so have efforts to improve data collection, analysis and modelling, and thereby the efficiency of transportation systems. Advanced traveller information systems are now used in cities worldwide. Most of them are based on historical and real-time data, and post current travel-time estimations and future travel-time predictions both on the Web and on message signs at major urban arterials.
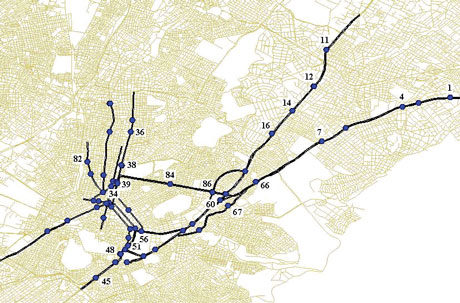
Practically every urban traveller information or traffic management system is based on traffic data collected by point sensors (eg single or double loop detectors, overhead video cameras). These provide estimates of flow, volume, occupancy and speed, averaged over time intervals that vary from thirty to ninety seconds. Part of the point-sensor data is usually calibrated with the use of floating car data. Figure 1 depicts a sample from a set of approximately 500 point sensors located in the Athens (Greece) road network. Representative subsets of the database provided by these sensors have been used in testing most of the statistical models developed in RAD.
Unfortunately, most of the point sensors employed worldwide are single loop detectors. These can only estimate traffic volume and occupancy and do not directly measure velocity, the most useful variable for traffic control and traveller information systems. Researchers in RAD are using recently developed methods that estimate velocity from single loop data: like a number of related methods these techniques are based on the preceding estimation of mean vehicle length. Moreover, to correct for unstable speed behaviour, especially during light traffic, smoothing spline algorithms are applied before travel-time estimations (Figure 2, left). To identify locations with similar traffic patterns, curve-clustering methods are applied at an exploratory stage (Figure 2, right). Holes in the data due to missing or bad observations are filled with imputed values; for that purpose, the nonlinear space-time models originally developed for forecasting are employed.
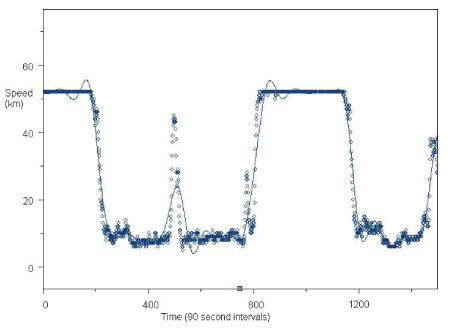
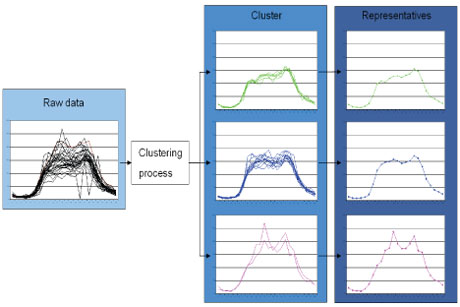
Perhaps the most challenging statistical issue in advanced traveller information systems is travel-time forecasting. Researchers in RAD are pioneers in the development and application of nonlinear space-time models for speed forecasting, and their models display a forecasting performance superior to existing methods. Models are spatial in the sense that they incorporate information related to the road network topology by using suitably defined spatial weight matrices. Models are nonlinear in the sense that relationships between upstream/downstream locations are not constant but are allowed to vary according to time and traffic state (free-flow/congestion). For instance, during free flow an upstream detector may have better predictive power than a downstream one, while the opposite may be true during congestion. Forecasting models are also nonlinear in the sense that error variance is not considered constant: separate autoregressive models provide variable confidence intervals for the forecasts based on time and traffic state.
The current research interests of the RAD group focus mostly on the application of the aforementioned methods to a new traffic information system for Athens. The system will provide real-time information via the Web and cellular phone services. The research is partially supported by LIAISON, a 42-month project co-funded by the European commission under the 6th Framework Programme and led by Alcatel Alenia Space.
Links:
http://www.iacm.forth.gr/regional/
http://liaison.newapplication.it
Please contact:
Poulicos Prastacos
IACM-FORTH, Greece
Tel: +30 2810 391767
E-mail:poulicosiacm.forth.gr