by Sergio Ilarri, Dragan Stojanovic and Cyril Ray
Smart Cities depend on information regarding moving objects (e.g., people, vehicles, assets, etc.) being processed. We propose a framework to enable a fully-fledged semantic management of moving objects that can be efficiently and flexibly exploited in Smart Cities.
The different types of moving objects in a city, such as people, vehicles and assets, that are equipped with mobile devices (e.g., smartphones, wearable devices, smart sensors, etc.) have increasing computing, communication and sensing capabilities. Thus, they will play a key role in Smart Cities. The efficient management of the information generated, provided and used by these objects (including information on their locations, trajectories, features, behaviors, activities and environmental contexts) facilitates the improved understanding and analysis of how a city performs. In addition, this type of information assists in the goal of providing citizens with contextual and adapted services in a range of areas including traffic management, urban dynamics analysis, ambient assisted living, emergency management, and mobile health. Whilst significant effort has been invested in modelling and managing moving objects, and some progress has been made regarding the representation of semantics associated with them, further efforts are needed to achieve a fully-fledged semantic management approach for moving objects that can be efficiently and flexibly exploited.
By integrating methods and techniques developed in different fields, including moving object databases, spatio-temporal data mining, and the Semantic Web, it is possible to enhance the way moving object information is managed (with respect to the modelling, querying, processing and analysis components). For example, recent proposals claim that linking Location-Based Services (LBSs) and semantics can provide interesting benefits [1], such as flexible querying, management of semantic locations and trajectories, interoperability among different LBSs and providers, protection of personal location information, and reasoning in complex and dynamic contexts. Our idea is to develop and provide LBSs that understand the user’s requests and interactions, implicitly based on the semantics of mobility and contextual information, and know how to behave and adapt in dynamic and unexpected situations.
Incorporating semantics in such an Internet of (Moving) Things provides valuable semantic information and services to mobile users, thus supporting enhanced mobility in dynamic environments such as those found in Smart Cities. However, there are numerous research challenges that need to be adressed to make this idea a reality. The first lies in the collaborative collection of the data required (e.g., the data measured by sensors on the moving objects). The data collection can be achieved through participatory/ collaborative sensing but this is subject to difficulties related to the correlation and analysis of those data, especially when this analysis is performed in a distributed way on mobile devices. Another challenge relates to the semantic representation of moving objects, where a unified approach that takes all the mobility aspects of moving objects (i.e., their trajectories, contexts, activities, goals, the physiological status of the user, environment, and the accessible services) into account, at different levels of granularity, is still missing. Thirdly, while interesting work has progressed on context-awareness, there is still a need for efficient integrated approaches for query processing in mobile environments, reasoning, and semantic searches, as well as an appropriate abstraction layer that enables the exploitation of the available functionalities. The fourth challenge relates to the inference of higher-level semantics (such as group mobility behaviours) from a large dataset of individual semantic mobility data and trajectories. Finally, we have to mention the problem of privacy protection. This is a particularly critical issue in Smart City environments, as the basic movement data is enhanced with rich semantics of the participating moving objects and their trajectories. Thus, it should be preserved according to the required privacy preferences of the participating users.
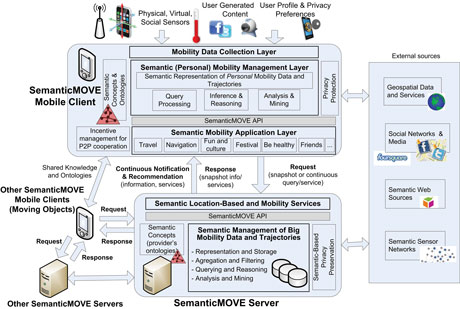
Prompted by the COST Action IC0903 on knowledge discovery from moving objects, we have collaboratively designed a generic and scalable distributed framework (SemanticMOVE), whose realization will enable the comprehensive management of the semantics of moving objects. Achieving this will leverage increased sensing, processing, interaction and communication capabilities in mobile devices in a scalable and effective way (Figure 1). As opposed to other related work, we envisioned a quite generic architecture which supports a fully distributed and interoperable scenario for the management of semantics of moving objects. Thus, each moving object can collect, store and analyze its own semantic mobility data and trajectories, and reason over them locally. It can share and exchange semantic mobility data and semantic concepts/knowledge (e.g., regarding the location, personal and social status, vehicle conditions, activities, behaviours, environment and traffic conditions, air pollution levels, etc.), with other moving objects/users/services in the vicinity, over ad-hoc wireless networks. It can also access and share information and knowledge through geo-social networks, social media services, and geospatial information services. Our framework includes fixed servers as an additional element of an ecosystem where the distributed and ad-hoc cooperation among moving objects, encouraged by some incentive mechanisms, plays a key role.
We believe that the development of such an approach will facilitate the deployment of interesting semantic mobility applications for Smart Cities. Whilst there are still some significant research issues that require further investigation, the initial results represent promising steps towards the intended direction [2, 3].
Links:
MOVE: Knowledge Discovery from Moving Objects: http://dmsl.cs.ucy.ac.cy/projects/cost/index.html
SemanticMOVE: http://webdiis.unizar.es/~silarri/SemanticMOVE/
References:
[1] S. Ilarri et al.: “Semantics in Location-Based Services - Guest Editors’ Introduction for Special Issue”, IEEE Internet Computing, ISSN 1089-7801, 15(6): 10-14, IEEE Computer Society, 2011
[2] B. Predic ,D. Stojanovic: “Localized Processing and Analysis of Accelerometer Data in Detecting Traffic Events and Driver Behaviour”, Journal of Universal Computer Science, ISSN 0948-695x, 18(9): 1152-1176, 2012
[3] I. Afyouni, C. Ray, C. Claramunt: “Spatial Models for Context-Aware Indoor Navigation Systems: A Survey”, Journal of Spatial Information Science, ISSN, 4(1): 85-123, 2012.
Please contact:
Sergio Ilarri
University of Zaragoza, Spain
E-mail:
Dragan Stojanovic
University of Nis, Serbia
E-mail:
Cyril Ray
Institut de Recherche de l’École Navale (IRENav), France
E-mail: