by Marie-Colette van Lieshout
Arak and Surgailis [1] introduced a class of random mosaics with remarkable mathematical properties. A collaborative project between researchers in Poland and at CWI shows that such models are useful for intermediate level image analysis because they can capture global aspects of an image without requiring a detailed description of the objects within it.
In a research project carried out in the context of the ERCIM MUSCLE Working Group, researchers at CWI and the Nicolaus Copernicus University in Toruń, Poland, studied random mosaic models[1] for intermediate level image analysis. These elegant mathematical models, which possess appealing properties such as consistency, have not been used in practice for a long time. The main deterrent to their use has been a naive Monte Carlo algorithm which iteratively makes small local changes to model realizations, converges slowly, and is difficult to program. The overall aim of our research project was to demonstrate that Arak-Surgailis type models can be applied by developing efficient Monte Carlo samplers. Initially, most attention was paid to segmentation and foreground/background separation [2]. More recent research at CWI has been focusing on extraction of fields in rural landscapes [3].
Figure 1, obtained from the collection of publicly released Synthetic Aperture Radar images at the NASA/JPL web site http://southport.jpl.nasa.gov, shows an agricultural region in Ukraine. A pattern of fields separated by tracks is visible, broken by some hamlets. Note that the tracks that run between adjacent fields show up in the image as whitish lines against the darker fields. In other words, a track is associated with a high image gradient. The objective is to extract the mosaic of fields.
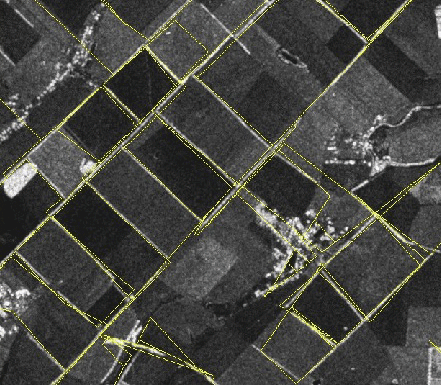
To do so, we build a family of straight lines on which to draw mosaics by computing the gradient of the data image (after convolution with a radially symmetric Gaussian kernel to suppress noise) and select the most salient edges. To quantify how well a particular mosaic fits the data, we use the integrated absolute gradient flux along its edges (thresholded to discourage spurious ones). Note that as a sum of contributions from each edge, this function is local in nature, which is convenient from a computational perspective.
To extract the fields, we use simulated annealing with a geometric cooling schedule. The result is shown in Figure 1 by the yellow lines. Note that all fields bordered by tracks are detected. A few false positives occur near the hamlets and are connected to the track network. The precision of the line placement is clearly linked to that of the underlying edge set. For example, the border of a field near the middle of the top of the image is misplaced. Naturally, one could expand the collection of edges to increase the precision, but this would incur a higher computational cost.
From a mathematical point of view, we introduce consistent random mosaics with polygonal tiles that are not necessarily convex, thus allowing a wide variety of shapes. The construction is inspired by the Arak-Surgailis model and extends our previous work [3]. We give an equivalent construction in terms of an interacting particle system that can be used to prove basic model properties including local and spatial Markovianity. This dynamic representation is also the foundation on which to build efficient Monte Carlo samplers that combine local modifications which are useful for fine tuning with global changes that allow fast exploration of the space of mosaics.
This research was funded by the EC 6th Framework Programme, as well as the Polish and Dutch Research Councils.
Full details of the research can be found in [2].
Link:
http:/homepages.cwi.nl/~colette
References:
[1] T. Arak, D. Surgailis: “Markov fields with polygonal realizations”, Probab. Theory Related Fields 80
[2] T. Schreiber, M.N.M. van Lieshout: “Disagreement loop and path creation/annihilation algorithms for binary planar Markov fields with applications to image segmentation”, Scand. J. Statist. 37
[3] M.N.M. van Lieshout: “Discrete multi-colour random mosaics with an application to network extraction”, Scand. J. Statist., to appear.
Please contact:
Marie-Colette van Lieshout
CWI, The Netherlands
Tel: +31 20 5924008
E-mail: