by Spiros Fotopoulos and Dimitrios Kastaniotis
Neurological disorders can be reliably assessed using the low-cost Microsoft Kinect depth sensor to record human gait, coupled with our Kinect Assessment Disorders (KAD) system to process the information.
{jcomments on}In recent years, computer science researchers, keen to develop more natural and non-intrusive means of human-machine interaction, have become interested in analysing human motion. Although some impressive methods have been proposed, the integration of such systems has been stalled by several factors: in particular the variability in scale, colour and texture of the captured scenes makes the problem almost intractable.
Recently, with the launch of low cost depth sensors, such as Microsoft’s Kinect, the landscape of Human Machine Interface (HMI) has started to change. This breakthrough in the history of human-machine interaction has triggered the design of new algorithms both for the task of pose estimation in real time and for extracting high level information from actions.
Beyond the area of HMI, Kinect also finds applications in human body gait analysis [1], for example, recent research has shown that gender recognition is feasible using recordings from the Kinect sensor [2] by utilizing the poses estimated by the Kinect SDK pipeline together with a novel feature representation. Thus we took advantage of the Kinect sensor’s capabilities in order to design novel techniques for processing human motion with the aim of assessing the state of patients with multiple sclerosis (MS).
Inspired by recent advances, our team, in collaboration with the Patras University Hospital (Department of Neurology), has been collecting data from patients diagnosed with MS. Using a special arrangement of Kinect sensors and a specialized computer program based on the Microsoft’s Kinect SDK, we are recording depth data, as well as the skeletal poses estimated by the Microsoft SDK pipeline. The experimental setup is located at the University Hospital of Patras, where a number of patients are being recorded every week following a purpose-designed protocol.
Our team is focusing on the development of “KAD”, a novel computer aided diagnosis system that can learn how to distinguish between different populations of patients by imitating a human expert’s ability to assess gait. This can be achieved, for example, by applying the “two minute test”, the established method used by specialists. This project started in early 2012, and it is expected to conclude by the end of 2015.
In order to distinguish between different populations of MS patients, we utilize state of the art pattern recognition techniques [3] that are appropriate for applying to a small number of samples per subject (or class) and that have been proven to work in similar tasks [3]. Our initial work on action recognition and gait-based gender recognition [2] already shows promising results. Namely, we have already proposed methods for capturing the dynamical characteristics of human motion by encoding the eight skeletal primitives depicted in Figure 1, using Euler angular representation. This results in the trajectories depicted in Figure 2, which seem to be very efficient at capturing the intrinsic parameters of human motion. These results lend strength to our hypothesis that intrinsic parameters of human gait can be captured, and this ability may allow us to differentiate among several types and states of MS patients.
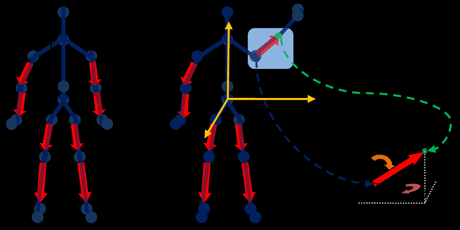
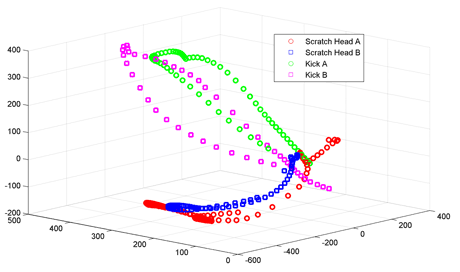
Our project belongs to the field of Computer Assisted Diagnosis. In the future, we plan to extend KAD to other kinds of neurological disorders that affect human motion. In particular we plan to focus on the early diagnosis of several neurological diseases by using videos captured from a consumer device (Kinect) or even from gait sequences recorded in a laboratory.
References:
[1] M. Gabel et al: “Full body gait analysis with Kinect”, Engineering in Medicine and Biology Society (EMBC), 2012 Annual International Conference of the IEEE, pp.1964-1967, 2012
[2] D. Kastaniotis, et al: “Gait-based Gender Recognition using Pose Information for Real Time Applications”, DSP 2013, in press
[3] Theodorakopoulos, et al: “Pose-based Human Action Recognition via Sparse Representation in Dissimilarity Space”, J. Vis. Commun. Image R. (2013).
Please contact:
Spiros Fotopoulos
University of Patras, Greece
Tel: +30 2610 99 6058
E-mail: