by Franco Alberto Cardillo, Giuseppe Amato and Richard Connor
Biological models of the human visual system can be exploited to improve the current state of the art in Content-Based Image Retrieval (CBIR) systems.
{jcomments on}We focus on novel ways to include local image descriptors, such as SIFT (scale invariant feature transform) [1] and SURF (speeded up robust features), in Content-Based Image Retrieval (CBIR) systems. Since there can be thousands of SIFT keypoints in a single image, their number needs to be reduced in order to use them in a CBIR context. We use a computational model of the human visual attention system to compute image saliency and restrict the comparisons among images to their most salient regions. The computational model of bottom-up attention is an extension of a well-known model by Itti et al. [2].
Given an image as a query, a CBIR system returns a set of images ranked according to their visual similarity to the query image. Classical approaches to CBIR use global image features, ie features whose values depend on the overall appearance of the image. However, global features yield poor results on semantic queries where the user is not interested in the overall image but in certain sub-images containing particular objects.
The basic assumption behind our work is that a user selects a query image mainly by looking at its most salient areas, and thus only the most salient image areas should be compared by the similarity function. In order to compute the image saliency we implemented a biologically-inspired and biologically-plausible computational model of the human visual attention system. The model encodes the input image according to what is known about the human retina and the first stages of the higher visual system.
When we open our eyes we see a colourful and meaningful three-dimensional world. This visual experience starts in our retina where the light strikes our photoreceptors, which are connected to ganglion cells through an intermediate layer of bipolar cells. Starting from the photoreceptors, the full visual experience is built up: features, such as intensity and colours, are extracted; lines with various orientations are detected; and the information flow is routed to the correct higher level neural circuits. The basic encoding performed at these early visual stages has a centre-surround organization: cells give a strong output when the centre of their receptive field contains their preferred stimulus, while the surrounding region does not (or vice-versa). Furthermore, the size of the receptive fields increases when moving from the centre to the periphery of the retina.
In our model, the input image is encoded in the Lab colour space, with the three channels L, a, and b encoding, respectively, the lightness, the red-green, and the blue-yellow opponent channels. The Lab values are then split into five different channels: intensity, red, green, blue, and yellow. These channels are used to build centre-surround (eg, red-on/green-off) feature maps, as found in the primate retina. Oriented features are extracted using Gabor filters with parameters set to values respecting the biological responses in the V1 cortical area. All the channels are encoded using Gaussian pyramids to mimic a space-variant retinal organization and introduce scale invariance into the model. The feature maps in the various dimensions (colour, intensity, oriented features) are merged into feature conspicuity maps encoding the contribution that the individual feature maps provide to the overall saliency. The feature conspicuity maps are then integrated into local saliency maps (one map per level of the Gaussian pyramid), which are finally merged into a global saliency map (see example in Figure 1).
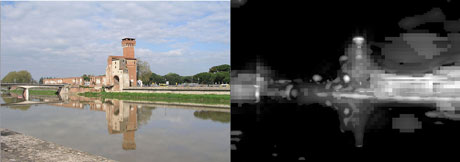
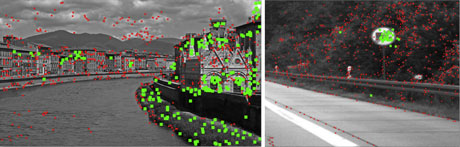
We experimented with two different tasks using two well-known and publicly available datasets: the Pisa-Dataset for landmark recognition and the STIM dataset for object recognition. The two sets were split into a training set and a test set. We extracted both SIFT keypoints and the saliency maps from each image in the two sets. Image matching was performed by comparing the keypoint descriptors in two images and searching for matching pairs. The candidate pairs for the matches are verified to be consistent with a geometric transformation using the RANSAC algorithm [3]. The percentage of verified matches is used to determine whether or not the two images contain the same rigid object. For each test image, the best candidate among the images in the training set is selected and its label is assigned to the test image.
We compared the results with and without the saliency filtering. When using the saliency map to filter out the keypoints, we applied several thresholds in order to keep areas at various degrees of saliency. The results are very promising. On the two datasets, the accuracy improves or just slightly decreases while the processing time is drastically reduced. For example, on the Pisa-Dataset a single test image is processed in 7.2 seconds on average, and in under one second when either all the keypoints or only 30% of those belonging to image areas with high saliency are kept.
In the future, we plan to experiment with larger datasets. For this purpose, we have currently implemented a parallel version of the visual attention model and extracted the saliency maps from the MIRFLICKR dataset containing one million images (using the HECToR supercomputer at the University of Edinburgh - HPC-EUROPA2 project.
This research is conducted as part of a joint collaboration involving research groups at the Institute of Information Science and Technologies of the Italian National Research Council, and the Department of Computer and Information Sciences of the University of Strathclyde, UK.
References:
[1] Lowe, D.G., “Distinctive image features from scale-invariant keypoints”, IJCV 3(1), 2004.
[2] Itti, L. et al., “A model of saliency-based visual attention for rapid scene analysis”, PAMI 20(11), 1998.
[3] Fischler, M.A., Bolles, R.C., “Random sample consensus: A paradigm for model fitting with applications to image analysis and automated cartography”, Commun. ACM, 24(6), 1981.
Please contact:
Franco Alberto Cardillo
ISTI-CNR, Italy
E-mail: