by Grégory Rogez, Deva Ramanan and J. M. M. Montiel
Camera miniaturization and mobile computing now make it feasible to capture and process videos from body-worn cameras such as the Google Glass headset. This egocentric perspective is particularly well-suited to recognizing objects being handled or observed by the wearer, as well as analysing the gestures and tracking the activities of the wearer. Egovision4Health is a joint research project between the University of Zaragoza, Spain and the University of California, Irvine, USA. The objective of this three-year project, currently in its first year, is to investigate new egocentric computer vision techniques to automatically provide health professionals with an assessment of their patients’ ability to manipulate objects and perform daily activities.
Activities of daily living (ADL) represent the skills required by an individual in order to live independently. Health professionals routinely refer to the ability or inability to perform ADL as a measure of the functional status of a person, particularly in regards to the elderly and people with disabilities. Assessing ADL can help: 1) guide a diagnostic evaluation, 2) determine the assistance a patient may need on a day-to-day basis or 3) evaluate the rehabilitation process. Initial deployment of technologies based on wearable cameras, such as the Microsoft SenseCam (see Figure 1a) have already made an impact on daily life-logging and memory enhancement. We believe that egocentric vision systems will continue to make an impact in healthcare applications as they appear to be a perfect tool to monitor ADL. One unique wearable camera can potentially capture as much information about the subject's activities as would a network of surveillance cameras. Another important benefit is that the activities are always observed from a consistent camera viewing angle, ie in first-person view.
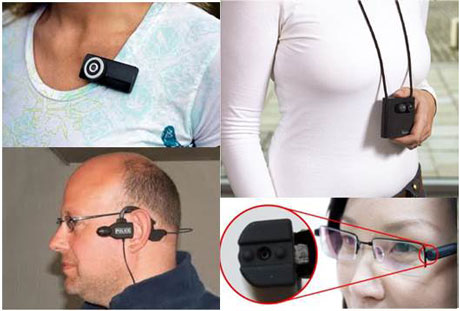
![Figure 1 a (top) Examples of wearable cameras (Clockwise from top-left) lapel, neck-worn Microsoft SenseCam, glasses and head-worn camera. Figure 1b (bottom) Example of a processed image from [1]. Figure 1 a (top) Examples of wearable cameras (Clockwise from top-left) lapel, neck-worn Microsoft SenseCam, glasses and head-worn camera. Figure 1b (bottom) Example of a processed image from [1].](/images/stories/EN95/rogez1b.jpg)
Recent work on ADL detection from first-person camera views [1] (Figure 1b) demonstrated an overall performance of 40.6% accuracy was obtained in ADL recognition, and 77% when simulating a perfect object detector. In egocentric vision, objects do not appear in isolated, well positioned photos, but are embedded in a dynamic, everyday environment, interacting constantly with one another and with the wearer. This greatly complicates the task of detection and recognition, especially when an object is being manipulated or occluded by the user's arms and hands.
EgoVision4Health is addressing this problem. Our work is organized along three research objectives: 1) to advance existing knowledge on object detection in first-person views, 2) to achieve advanced scene understanding by building a long-term 3D map of the environment augmented with detected objects, and 3) to analyse object manipulation and evaluate ADL using detailed 3D models.
The analysis of “near-field” object manipulations - and consequently ADL recognition and assessment - could benefit greatly from having all the objects that are likely to be manipulated already located in the 3D environment. For example, if we want to determine whether a person is picking up a mug the wrong way due to an injury it seems important to know where the handle of the mug is, and how it is oriented in 3D. Another advantage of having all the objects already located around the subject is that we can categorize the scene and improve ADL recognition, eg cooking only happens in the kitchen. For a real breakthrough in ADL detection and assessment from a wearable camera, a thorough a priori understanding of the subject’s environment is vital.
Since we expect Kinect-like depth sensors to be the next generation of cheap wearable cameras, we use a RGB-D camera as a new wearable device and exploit the 2.5D data to work in the 3D real-world environment. By combining bottom-up SLAM techniques and top-down recognition approaches, we cast the problem as one of “semantic structure from motion” [2] and aim at building a 3D semantic map of the dynamic environment in which the wearer is moving. We plan to model objects and body parts using 3D models and adopt the completely new approach of considering each ADL as the interaction of the 3D hands with 3D objects.
The hypothesis at the basis of our proposal is that the EU’s well-established technology in mapping for robotics and the latest computer vision techniques can be cross-fertilized for boosting egocentric vision, particularly ADL recognition. Our goals are motivated by the recent advances in object detection, human-object interactions and ADL detection [1] obtained by UC Irvine’s group, as well as by the expertise of the University of Zaragoza in robust and real-time Simultaneous Localization and Mapping (SLAM) systems [3].
The tools currently available in each of these domains are not powerful enough alone to account for the diversity and complexity of content typical of real everyday life egocentric videos. Current maps, composed of meaningless geometric entities, are quite poor for performing high-level tasks such as object manipulation. Focusing on functional human activities (that often involve interactions with objects in the near-field), and consequently on dynamic scenes, adds to the challenging and interesting nature of this problem, even from a traditional SLAM perspective. On the other hand, ADL detectors perform poorly in the case of small objects occluded by other surrounding objects or by the user's body parts. Research breakthroughs are thus required, not only in vision-based ADL recognition and SLAM, but also in exploiting the synergy of the combination.
EgoVision4Health is financed by the European Commission under FP7-PEOPLE-2012-IOF through grant PIOF-GA-2012-328288.
Links:
http://www.gregrogez.net/research/egovision4health/
http://cordis.europa.eu/projects/328288
References:
[1] H. Pirsiavash, D. Ramanan: “Detecting activities of daily living in first-person camera views”, in proc. of IEEE CVPR, 2012, pp. 2847-2854
[2] N. Fioraio, L. Di Stefano, “Joint Detection, Tracking and Mapping by Semantic Bundle Adjustment”, in proc. of IEEE CVPR, 2013, pp. 1538-1545
[3] J. Civera, A. J. Davison, J. M. M. Montiel: “Structure from Motion using the Extended Kalman Filter”, Springer Tracts in Advanced Robotics 75, Springer 2012, pp. 1-125.
Please contact:
Grégory Rogez
Aragon Institute for Engineering Research (i3A),
Universidad de Zaragoza, Spain
E-mail: