by Paula Crăciun and Josiane Zerubia
Earth observation satellites represent a significant resource when it comes to acquiring data about the Earth. Satellite data is used in a range of fields, including environmental monitoring, map updating and meteorology. Since the launch of the first Earth observation satellite, the resolution of the optical sensors installed on board has greatly improved, thus, nowadays, panchromatic images can be acquired at a resolution equal or lower than 0.7 [m] (ie GeoEye, Pleiades). This makes it possible to recognize small objects, such as boats and cars.
When it comes to the problem of object extraction, two conceptually different techniques are usually used. The first, a pixel-based approach, takes only the spectral information of individual pixels into account. It has proved its usefulness in the case of low or medium resolution imagery, but has found its limits in high resolution imagery owing to the rich information content in such data. The main difficulties appear when trying to cope with objects that are unevenly illuminated, partially occluded or blended with the background. The second type of technique considers objects, rather than individual pixels during the extraction. It also takes shape and textural information into account, along with the spectral data.
A possible framework for object-based solutions is offered by stochastic geometry. The quest is to find a pattern of objects that fits the image, which is considered to be a realization of a random process. Three main ingredients are needed to build a spatial pattern analysis tool: a prior to give a general aspect of the searched solution, a conditional probability that depends on the data, and an optimization method to get the best solution to the problem which consists of maximizing a probability or minimizing an energy function [1].
In this regard, statisticians working in image processing have introduced marked point processes as a tool to solve pattern analysis problems. By developing specialized models, different kinds of objects in complex scenes can be handled. The marked point processes are used as priors. Interactions between objects are defined, allowing for the incorporation of global properties into the model. The shape of the objects is modelled by constraints on the marks of the point process.
The conditional probability depending on the data is given by the likelihood that a configuration of objects fits the actual image. Finally, by maximizing a Bayesian criterion, such as the Maximum A Posteriori using an optimization method, one obtains the resulting configuration, eg the configuration which maximizes the product between the prior and the likelihood.
One particularly complex case is the extraction of boats in harbours. The objects of interest are usually clustered around the docks, vary in size and orientation, and tend to be very close to each other. Here, objects are modelled as stochastic ellipses. Constraints on the prior do not allow for object overlapping and neighbouring objects should have similar orientations. The conditional probability depending on the data is determined individually for each object in a given configuration by computing a contrast distance between the interior of the ellipse and part of its surrounding background.
Additional prior information is embedded into the model by identifying the local orientations of the objects [2]. By determining how each dock in the harbour is oriented, it is possible to make inferences about the orientation of the boats. Figure 1 shows results for boat extraction in a harbour.
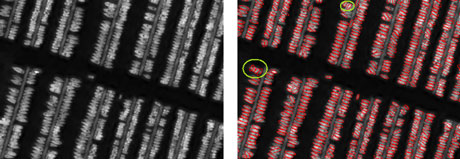
The areas highlighted in green present extraction errors due to the fact that the objects in the image are larger than the sizes specified in the model.
Marked point processes offer a reliable tool and moreover, a general framework for extracting objects from high resolution optical satellite images. Their usability in dynamic images (ie for object tracking) is currently being investigated.
Link: https://team.inria.fr/ayin/
References:
[1] S. Ben Hadj, F. Chatelain, X. Descombes and J. Zerubia, “Parameter estimation for a marked point process within a framework of multidimensional shape extraction from remotely sensed images”, Proc. ISPRS, XXXVIII, 2010
[2] P. Craciun and J. Zerubia, “Unsupervised marked point process model for boat extraction in harbors from high resolution optical remotely sensed images”, Proc. IEEE ICIP, 2013
Please contact:
Josiane Zerubia,
AYIN research team, Inria, France
E-mail: