by Adrien Gaidon, Zaid Harchaoui and Cordelia Schmid
Automatic video understanding is a growing need for many applications in order to manage and exploit the enormous – and ever-increasing – volume of available video data. In particular, recognition of human activities is important, since videos are often about people doing something. Modelling and recognizing actions is as yet an unsolved issue. We have developed original methods that yield significant performance improvements by leveraging both the content and the spatio-temporal structure of videos.
Video is a popular medium, spanning a wide range of sources and applications. One of the main challenges is how to easily find meaningful content, for instance, particular events in video-surveillance data and video archives; certain types of human behaviour for autonomous vehicles in the area of robotics; and gestures in human-computer interfaces.
Owing to the overwhelming quantity of videos, answering this question calls for tools that can automatically analyse, index, and organize video collections. The problem is, however, that computers are currently unable to understand what a video is about. The relationship between the bits composing a video file and its actual information content is complex.
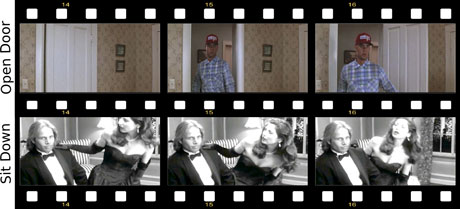
Our research focuses on designing computer vision and machine learning algorithms to automatically recognize generic human actions, such as “sitting down”, or “opening a door” (see Figure 1), which are often essential components of events of interest. In particular, we investigate the fundamental structure of actions and how to use this information to accurately represent real-world video content [1].
The main difficulty lies in how to build models of actions that are information-rich, in order to correctly distinguish between different categories of action (such as running versus walking), while at the same time being robust to the large variations in actors, motions, and imaging conditions present in real-world data.
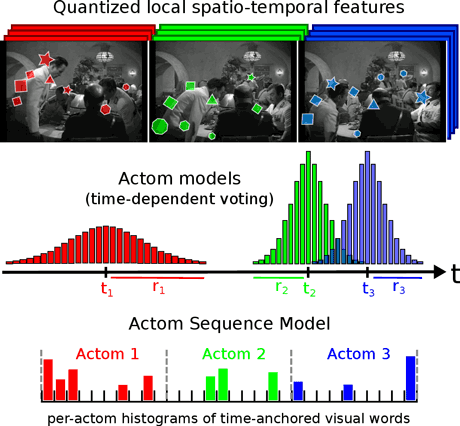
We discovered that many types of action can actually be modelled as a simple sequence of action atoms, or “actoms”, which correspond to meaningful atomic temporal parts [2]. We found a robust parameterization, our “Actom Sequence Model” (see Figure 2), which efficiently leverages an action’s temporal structure. Our method allows for more accurate action detection in long video sequences, which is particularly important when aiming to index events in large video archives such as the BBC motion gallery or the French INA repositories.

The previous approach is well suited to actions performed in a few seconds. Some activities, however, are not just a short sequence of steps, but have a more complex spatio-temporal structure, for instance sports activities like weight-lifting (see Figure 3). In these cases, we found that it is possible to extract elementary motions and how they relate to each other without human intervention [3]. This allowed us to represent complex activities using a tree-like data structure that can be efficiently computed and compared across videos. We showed that our approach can tag high-level activities in sports videos, movies, TV archives, and internet videos.
In all cases, we conducted thorough experiments on real-world videos from a wide array of sources: movies, TV archives, and amateur and internet videos (YouTube). We showed that our methods outperform the current state of the art on a variety of actions. These promising results suggest that our structured models could be applied in many different application contexts, for instance to recognize complex behaviours of autistic children for diagnostic assistance, to automatically browse video archives based on their contents instead of their meta-data, or to index web videos by generating video tags based on detected events.
This research was conducted during the PhD thesis of Adrien Gaidon, under the supervision of Zaid Harchaoui and Cordelia Schmid, at the joint Microsoft Research – Inria research center in Paris, France, and with the LEAR team, Inria Grenoble, France.
References:
[1] A. Gaidon: “Structured Models for Action Recognition in Real-world Videos”, PhD thesis,
http://tel.archives-ouvertes.fr/tel-00780679/
[2] A. Gaidon, Z. Harchaoui, C. Schmid: “Temporal Localization of Actions with Actoms”, IEEE Transactions on Pattern Analysis and Machine Intelligence, 2013
http://hal.inria.fr/hal-00804627/en
[3] A. Gaidon, Z. Harchaoui, C. Schmid: “Recognizing activities with cluster-trees of tracklets”, British Machine Vision Conference, 2012, Guildford, United Kingdom.
http://hal.inria.fr/hal-00722955/en
Link:
https://lear.inrialpes.fr/people/gaidon/
Please contact:
Adrien Gaidon
Xerox Research Center Europe