by Loredana Murino, Umberto Amato and Bruno Alfano
Involvement and morphological changes of brain structures both in aging processes and in neurodegenerative diseases can be analysed using Magnetic Resonance imaging. Our aim is to automate the procedure through supervised brain tissue classification.
{jcomments on}Magnetic Resonance (MR) has proven to be one of the most promising diagnostic modalities for the study of the brain. This highly flexible technique makes it possible to examine the brain’s interior structures as well as its activity at rest or under specific stimuli.
Automatic tissue recognition (also known as segmentation) is an important and challenging task in the processing and analysis of brain MR images, and during the last decade a large number of software packages have been developed to classify the three major brain tissues: grey matter, white matter and cerebro spinal fluid.
There is a growing interest in understanding the involvement and the possible morphological changes of minor brain structures both in normal aging processes and in the presence of neurodegenerative diseases. While the segmentation of major tissues has been intensively investigated, our target is tissue classification and segmentation of subcortical structures for which only a few tools are available.
Our study is carried out at the Istituto per le Applicazioni del Calcolo “Mauro Picone” (IAC-CNR, Naples) in collaboration with the Istituto di Biostrutture e Bioimmagini (IBB-CNR, Naples) under the project MEdical Research in ITaly (MERIT) funded by the Italian Ministry of University and Research. It focuses on the classification of multispectral MR brain images using supervised methods. This requires a training data set (gold standard), comprising a set of voxels and corresponding intensities for which the tissue is “certainly” known. Classifiers learn characteristics of all tissues from this data set and they are then able to classify voxels of new data sets.
As a gold standard we have considered a recently developed three-dimensional digital brain phantom (DBP) [1]. The following 17 tissues are defined in the DBP: 11 intracranial tissues (gray matter, white matter, cerebro spinal fluid, pallidus, putamen, thalamus, caudate nucleus, red nucleus, dentate nucleus, substantia nigra, and intracranial connective), and five extracranial ones (fat, muscle, vitreous humor, extracranial connective, and extracranial fluid); a further class (LowPD) comprising intra and extra-cranial voxels characterized by a low proton density value is included. The model contains 256 x 256 x 150 near-isotropic 0.9375 x 0.9375 x 1 mm voxels; each voxel is labelled according to its assignment to a unique tissue. The corresponding simulated signals are provided in the form of T1W (510/15ms TR/TE) and PDW - T2W (1867/15-90ms TR/TE) axial slices.
In addition to providing the gold standard, the DBP model also serves as a reference to evaluate the performance of the classification methods.
We compared several classification methods belonging to the family of Discriminant Analysis (DA) (Principal (PCDA), Independent (ICDA) and Oblique (OCDA) Component Discriminant Analysis [2]), with two popular methods from the machine learning literature, namely, K-Nearest Neighbour (KNN) and Support Vector Machine (SVM).
These methods do not use spatial correlation in images, since they classify images voxel by voxel independently. As a consequence, the accuracy of the classification degrades and artefacts are present in classified images (eg, “salt and pepper” noise). Therefore spatial information has been incorporated into the classification scheme and quantitatively assessed. This has been accomplished largely by introducing local prior probabilities in Discriminant Analysis that take into account both the tissues present in the neighbourhood of each voxel and a probabilistic atlas [3] of all the intracranial brain tissues. In a first phase of the project, the training step was made on a subset of the gold standard and classification was performed on the remaining voxels. In a second phase we have considered 20 real datasets from normal volunteers. Each study consists of T1W, T2W and PDW axial slices covering the entire brain, acquired using a Philips Intera 1.5T MR scanner. The training step of classification is performed on full gold standard, whereas actual segmentation is performed on the real studies.
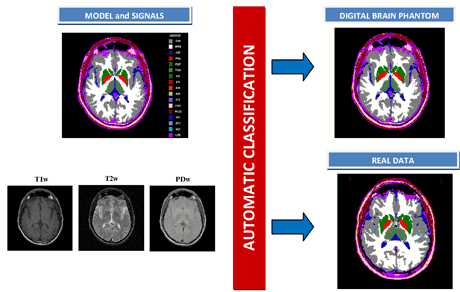
Figure 1 (left) shows a single slice of the digital brain phantom and the corresponding MRI T1W, T2W and PDW signals, whilst on the right the automatic tissue classification obtained applying the OCDA method both to the corresponding slice of the DBP dataset and to a single slice of one selected real study are shown. Inspection of the classification images and analysis of the numerical results (success percentage greater than 90% for both major tissues and subcortical structures) confirm that the supervised methods (in particular OCDA) are able to correctly classify the real studies.
Future work will focus on the classification of pathological studies with the fundamental objective of locating and monitoring lesions in brain tissues.
Link:
http://lab.ibb.cnr.it/
References:
[1] Alfano, B et al.: “An MRI digital brain phantom for validation of segmentation methods”, Med. Image Anal., 2011; 15(3):329–39.
[2] Selvan SE et al.: “Descent Algorithms on Oblique Manifold for Source-Adaptive ICA Contrast”, IEEE Trans. Neural Netw. Learn. Syst., 2012; 23(12):1930-47
[3] Mazziotta J. et al.: “A probabilistic atlas and reference system for the human brain: International Consortium for Brain Mapping (ICBM)”, Phil. Trans. R. Soc. Lond. B, 2001; 356(1412):1293–1322.
Please contact:
Loredana Murino, Umberto Amato
CNR -Istituto per le Applicazioni del Calcolo “Mauro Picone”, Italy
E-mail:
Bruno Alfano
CNR- Istituto di Biostrutture e Bioimmagini, Italy
E-mail: