by Katarzyna Wac
Quality of Service Information System (QoSIS) focuses on measurement-based performance evaluation of wireless access networks provided by diverse mobile network operators in diverse locations and times. We have developed an Android OS mobile application that uses measurement data provided by real mobile users living in the Geneva area to predict the networks' expected performance. Measurement data, and therefore predictions, are available for Swiss operators: Swisscom, Sunrise, Orange CH, as well as French operators: SFR, Bouygtel and Virgin.
The effectiveness of any mobile service depends on the quality of service (QoS) provided by the wireless access network it uses. However, the QoS is often unknown, as public and private wireless access network providers, mobile network operators (MNOs) for instance, tend to not disclose detailed, real-world QoS-information. For marketing purposes, these providers usually advertise only the best data rate values for their networks.
According to the 4G vision, in the near future wireless access networks of various providers - employing different wireless access technologies - will be ubiquitously available for mobile service users. Also, a seamless handover between these networks will support users’ mobility. Ideally, users should have a priori knowledge about the QoS provided by different networks. Based on that knowledge, a mobile device, on behalf of its user, could make an informed choice about which wireless access network provider and technology to use for the preferred mobile services of the user.
To date, there are no unbiased, external providers of such information in the mobile business landscape. Users, who gain personal experience of various mobile services, may eventually use their acquired knowledge to manually reconfigure their devices and share their knowledge with family and friends. However, there is no service platform enabling users to collaboratively share their collected knowledge.We are developing a Quality of Service Information System (QoSIS) to fill this gap. QoSIS distributes predictions to mobile devices about the QoS provided by the different wireless access networks available at a given geographical location and time. These predictions are then used by a mobile device, on behalf of the user, to choose a wireless access network provider and technology to be used. The knowledge furnished by QoSIS also allows mobile service providers to adapt their service delivery to the predicted QoS, thus increasing the service quality and improving user experience.
Mobile devices, on behalf of their users, can contribute to the QoSIS database in a collaborative information-sharing manner by submitting collected data about the QoS provided during their mobile service use, given the selected wireless access network provider and technology. The principal dimensions of the QoSIS database are: geographical location, time, wireless access network provider and wireless access technology. QoSIS is based on a QoS prediction engine. Based on machine learning algorithms, the engine builds a heuristic from which to derive predictions.
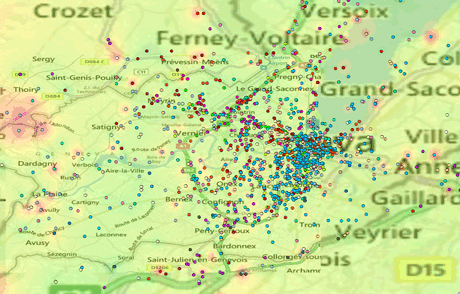
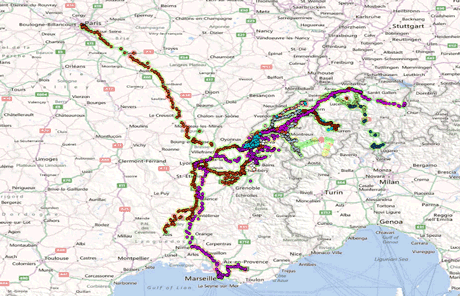
We have assessed the feasibility of deriving predictions in a case study based on delay measurement data collected from the mobile device of a health telemonitoring service user. We have shown that it is feasible to predict the value of delay for a device, based on its own measurement data or on data collected by another device being used at the same location and time and using the same wireless access network provider and technology. It is also feasible to predict the value of delay for a device, based on delay measurement data collected by both devices [1].
In contrast to existing approaches, the proposed QoSIS is entirely user-driven: mobile service users collaboratively create the QoS-information that would provide the basis for QoS prediction for other users. QoSIS therefore implements the Mobile Web 2.0 paradigm. In line with this approach, we have also assessed the business scenarios for an enterprise based on QoSIS services - provisionally named “QoSIS.net”. This enterprise could provide a QoS prediction service to its customers: mobile service providers and MNOs, as well as mobile service users. As part of the case studies, we have investigated business-to-business (B2B) and business-to-consumer (B2C) scenarios for QoSIS.net. We have shown that these scenarios are beneficial for all parties in terms of increased revenue, increases in mobile service quality and improvement of mobile user’s experience [2].
The Quality of Life technologies group at University of Geneva, Institute of Services Science, is developing the QoSIS.net project. Future activities include deployment of QoSIS.net in the mHealth area – benefiting mobile patients and their caregivers by providing QoS-assurance for health tele-monitoring and treatment services [3].
Links:
http://www.qosis.com/
http://www.qol.unige.ch/
References:
[1] K. Wac, M. Hilario, B.J. van Beijnum, et al: “QoS-Predictions Service: QoS Support for Proactive Mobile Services”, Wireless Network Traffic and Quality of Service Support: Trends and Standards, IGI Global Publisher, 2010
[2] K. Wac: “Collaborative Sharing of Quality of Service-Information for Mobile Service Users”, PhD thesis, Information Systems, June 2009, University of Geneva, Switzerland.
[3] K. Wac, M. Bargh, B.J. van Beijnum, et al: “Power- and Delay-Awareness of Health Telemonitoring Services: the MobiHealth System Case Study”, IEEE JSAC, Special Issue on Wireless and Pervasive Communications in Healthcare, 27(4): 525-536, IEEE Press, May 2009.
Please contact:
Katarzyna Wac
University of Geneva, Switzerland
Tel: +41 22 379 0242
E-mail: