by Pavel Vácha and Michal Haindl
Each type of wood has its own specific physical, aesthetic and economic properties; thus correct identification of wood species is required in numerous practical applications, from construction industry, manufacturing, furniture design, and restoration to pricing evaluation of wooden items. Fast, reliable, and practical recognition of wood species is therefore important, having potential impacts in a range of areas, including: the intended application, construction safety, and detecting illegal logging of endangered species. A mobile Android application that can automatically recognize wood species from a phone photo has been developed at the Institute of Information Theory and Automation AS CR in Prague..
The traditional method of identifying wood species involves manual browsing through digital wooden veneer catalogues and making a subjective judgement. This is labour intensive, and concentration problems can lead to errors. Additionally, gradual changes and changing shades due to variable light conditions are difficult for humans to detect. As an alternative, we aimed to develop an application to identify wood species using a smartphone camera, which returns the resulting species name and a corresponding high quality database wood specimen image. This computer-aided wood identification system retrieves a wood template from a digital wood database, selecting that which most closely resembles the query sample.
A wooden surface is captured by a smartphone camera with the developed Android application, and the image is transmitted to the server side which computes the advanced multispectral Markovian textural features [1] and finds the most similar wood species from its database. Markovian features are not only very efficient, compacting the representation of visual properties of wood, but are also invariant to illumination colour and robust to illumination heterogeneity and illumination direction, therefore the retrieval result is not influenced by illumination properties. The recognized wood species together with its high quality database pattern is sent back to the user so he or she can verify the classifier’s result. The challenging part of the method is to compare poor quality smartphone images taken under variable illumination and resolution conditions with high quality high resolution matte wooden textures stored in the wood database.
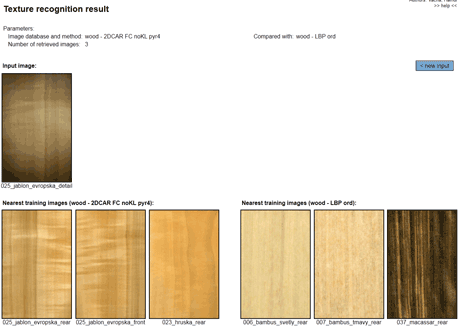
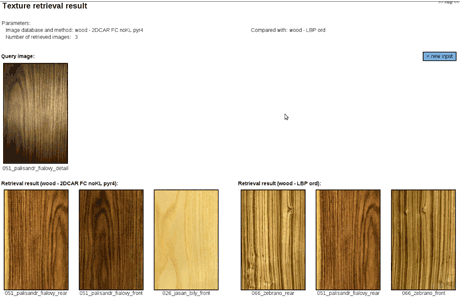
The performance of our application was verified on the wood database, which contains about sixty different European as well as exotic wood species, each with two sample images. Our Markovian textural features (2D CAR) [1] compared favourably with alternative Local Binary Patterns (LBP) [2] and Gabor textural features [3]. The test images were photographed from approximately the same distance and with camera oriented along tree rings to reduce variation in scale and rotation. These simple non-restrictive conditions were enough to raise the performance of the features above their rotation invariant versions. Our correct recognition rate was 66 %; LBP features 22 %, while the Gabor features completely failed due to non-homogeneous smartphone flash illumination. The wood recognition rate significantly improves (ie, Markovian features 81 %, LBP 55 %, Gabor features 48 %) if the input samples are measured with a high-end consumer digital camera. Although smartphone cameras have sufficient resolution (up to 10 mega pixels), their poor quality lenses and aggressive JPEG compression result in overall inferior image quality and thus a more demanding recognition task. Figures 1 and 2 illustrate the system’s typical performance applied to European and exotic wood samples.
References:
[1] P. Vácha, M. Haindl, T. Suk: “Colour and rotation invariant textural features based on Markov random fields”, Pattern Recognition Letters, vol.32, no.6, pp.771-779, 2011
[2] T. Ojala, M. Pietikäinen, T. Mäenpää: “Multiresolution gray-scale and rotation invariant texture classification with local binary patterns”, IEEE Trans. Pattern Anal. Mach. Intell. 24(7), 971–987 (2002)
[3] B. S. Manjunath and W. Y. Ma: “Texture Features for Browsing and Retrieval of Image Data”, IEEE Trans. on Pattern Anal. and Mach. Intelligence, 18(8), 1996.
Please contact:
Pavel Vácha, CRCIM (UTIA), Czech Republic
Tel: +420 2 6605 2365, E-mail: