by Martin Reczko, Panayiota Poirazi, Anastasis Oulas, Eleftheria Tzamali, Maria Manioudaki, Vassilis Tsiaras and Ioannis Tollis
Substantial advances in predictive, preventive and personalized (PPP) medicine are starting to emerge from computational simulations of complex networked models of metabolism ranging to the molecular level of detail. From the systems biology perspective of the digital patient, diseases are perturbations of biological networks through defective genes or environmental stimuli, and therapies are the interventions needed to restore these networks to their normal states. The Bioinformatics group at FORTH Heraklion is developing novel computational methods for identifying new parts of these networks both from genomic sequences and from metabolite time-series, and to generate meaningful visualizations of them.
Our bioinformatics activities are collaborations between the Biomedical Informatics Lab of the Institute of Computer Science and the Computational Biology Lab of the Institute of Molecular Biology and Biotechnology. The common goal is the data-driven discovery of novel regulatory networks. In most cases, these networks are related to various diseases. One specific focus is the study of the interferon signalling network and its interplay with clinically relevant pathogens such as Cytomegalovirus. In particular, we are developing computational methods to investigate the role in various diseases of a novel class of small regulatory genes called microRNA (miRNA).
Large parts of the general regulatory network operating with miRNAs are not yet known. We have therefore developed a computational pipeline to extract novel miRNAs from the human genome, using support vector machines trained on features of known miRNAs as the central classification method. In collaboration with the Universities of Pennsylvania and Toronto, we experimentally verified the actual expression of a large number of these miRNAs in many different human tissues. Subsequently, the regulatory function of these miRNAs can be partially predicted by computational methods in order to unfold the underlying regulatory network. To visualize these and other types of regulatory networks that contain genes annotated by the Gene Ontology project, we developed a visualization tool using circular drawings and treemaps (see Figure 1).
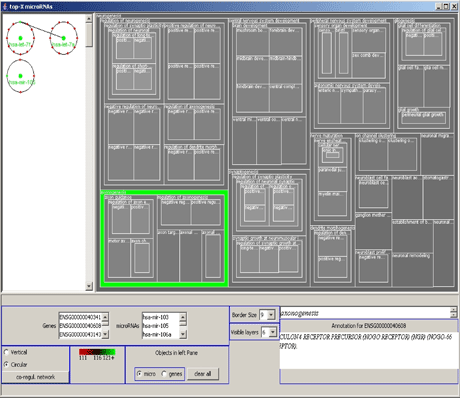
In developing methods to identify regulatory networks, we use time series of metabolite concentrations in yeast cells exposed to various types of environmental stress. At a higher level of detail we aim to identify the static modular organization of these networks, where the modules are defined as groups of co-regulated genes contributing to one specific biological function. We try to detect the kind of interrelations that govern each module, and the rules for interactions between modules. Initially we use gene expression data in conjunction with several statistical approaches, such as linear and higher-order correlation functions.
More accurate network models emulate the dynamic behaviour of all observable metabolites and have already been used to predict the presence of as yet unknown biological elements; for example, an unknown activating "modifier" in human colon carcinoma cells that might act as a novel therapeutic target. To derive these types of networks from time series we employ evolutionary optimization methods.
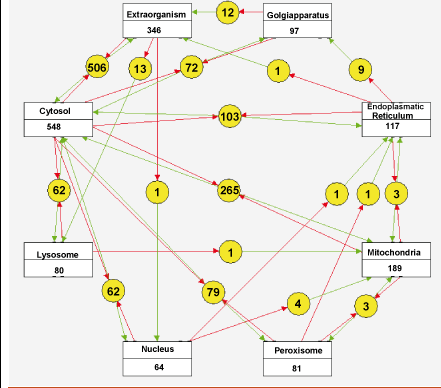
An example of the most successful multilevel model with a wide temporal and spatial range is the virtual heart that recently extended the spatiotemporal detail of the simulations to the level of fixed subcellular modules. Now the first in silico reconstructions of the complete human metabolic network have become available for this and many other predictive models for human diseases.
Figure 2 shows one possible visualization of the reactions in this network; we designed this using the Cytoscape tool. Apart from leading to fundamental advances in biology, these models will have a direct practical value in future medicine for the integration, analysis and classification of data.
This work is supported by the EU-funded projects INFOBIOMED and ACGT, and by the action 8.3.1 (Reinforcement Programme of Human Research Manpower). It is also assisted by the project PrognoChip, which is itself funded by the operational programme "competitiveness" of the Greek General Secretariat for Research and Technology.
Links:
http://www.ics.forth.gr/bmi
http://www.imbb.forth.gr/groups/computational.html
http://infobiomed.org
Please contact:
Martin Reczko
ICS-FORTH, Greece
E-mail: reczkoics.forth.gr