Big data research in Life Sciences typically focuses on big molecular datasets of protein structures, DNA sequences, gene expression, proteomics and metabolomics. Now, however, new developments in three-dimensional imaging and microscopy have started to deliver big datasets of cell behaviours during embryonic development including cell trajectories and shapes and patterns of gene activity from every position in the embryo. This surge of multicellular and multi-scale biological data poses exciting new challenges for the application of ICT and applied mathematics in this field.
In 1995, when I was in the early stages of my biology masters and starting to think about PhD opportunities, Nature published a short feature article entitled ‘The Boom in Bioinformatics’. The world needed bioinformaticians, the article said, “to take full advantage of the vast wealth of genetic data emanating from […] the Human Genome Project and other […] efforts.” With a combined interest in computer science and biology, you might have thought therefore, this promised a bright future for me. The problem was bioinformatics did not excite me. I didn’t believe it would solve the problem I had held closest to my heart since I had first seen tadpoles develop from eggs, embryogenesis. After all, embryos are not just bags of genes.
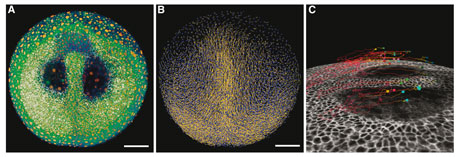
Figure: Zebrafish (Danio rerio) imaged live throughout early development (gastrulation). Snapshots of the tailbud stage. A: Raw data (fluorescent nuclei and membranes), display with avizo software, data cut at a depth of 100 microns. B: Display of detected nuclei and cell trajectories, calculated using the BioEmergences workflow (http://www.bioemergences.eu). A, B scale bar 100 microns. Close up in C to show selected clones (colored cubes) and their trajectories for the past 6 hours, in white an orthoslice of the membrane channel. Pictures by Nadine Peyriéras, BioEmergences.eu, CNRS Gif-sur-Yvette, France.
Technological developments in microscopy and image analysis are now producing a flood of new data that excites me much more. With this data, it is now possible to track the movements and behaviours of any cell, in an early embryo, organ, or tumour. With this capability we will now be able to identify what makes cells take a wrong turn in children with birth defects or how tumour cells can change their metabolism and movement to out compete their well-behaved neighbours and disrupt the structure and function of an organ. Such mechanistic insights will eventually make it possible to interfere with developmental mechanisms with a greater specificity than currently possible.
Conventional light microscopy can already follow the migration of a subset of individual cells (labelled with fluorescent markers) in organs but techniques are getting better. Two-photon microscopy techniques, used in conjunction with advanced image analysis, allow researchers to routinely generate all-cell datasets of developing embryos or organs. Applying this approach the BioEmergences platform at CNRS (Gif-sur-Yvette, France) recently produced a gene expression atlas featuring cellular resolution of developing zebrafish [1]. Soon we will be able to follow every cell in developing organisms and tissues and concurrently identify what genes they are expressing and what metabolites they are producing.
These developments will generate enormous new datasets that will be much bigger than those that currently exist in molecular biology. So how can we store such data in a structured way so that researchers can use it meaningfully to further the field of embryogenesis? First, the data must be stored in standard formats that facilitate sharing and make it accessible for on-going use after publication. For molecular data standards exist to ensure the gene sequence and functional features are captured. For example, Gene Ontology is a structured vocabulary that allows researchers to assign a readable list of well-defined biological functions to a gene. Two computer-oriented, domain-specific languages, SBML and CellML, can be used to describe the dynamic interaction networks of genes, proteins and metabolites. These languages create files that are similar to PDF files and they can be interpreted by many different software applications.
Ongoing initiatives in the field of information sciences are laying the foundations for similar data standards and domain-specific languages in the multicellular biology community. New versions of SBML will allow users to describe the distribution of molecules in fixed geometries and coupled cells. However, in a recent paper that proposed a Cell Behaviour Ontology (CBO) [2], it was argued that SBML is not the most efficient or insightful way to annotate embryological data. The multicellular organism is a collection of thousands to trillions of individual cells. Individually describing the gene expression levels and biophysical properties of each cell will create huge datasets but not necessarily yield useful insights. Even the most detailed three-dimensional movies or sets of cell trajectories are merely pretty pictures unless we can identify and label their components meaningfully. A useful comparison is thinking about the difference between providing a list of pixels in an image versus the list of things in that image. CBO focuses on describing the behaviour of cells and the dependency of those behaviours on the cell’s internal machinery. This includes its gene expression pattern and local environment. This declarative approach allows the CBO to categorise each cell in a developing embryo using a manageable set of cell types which range from the tens to hundreds in number. Each cell type is characterised by the same class of behaviours, thus, cells belonging to the same cell type share the same behaviours. Each cell follows a set of logical input and output rules that guide these behaviours and its transition from one cell type to another (i.e., differentiation). Many cell types in multicellular organisms are ‘sub-types’ whose behaviour varies in subtle ways around a general ‘base’ cell type. For example, the endothelial cells in a developing blood vessel are made up of two sub-types: ‘tip’ cells at the end of a sprouting blood vessel which are usually more spikey and motile and ‘stalk’ cells which occur to the back of the sprout. This approach allows the CBO to develop a hierarchical classification of cell types and cell behaviours.
Besides compressing the data, the classification of cell behaviours will also enable quantitative biologists to understand biological development to a point that, with the aid of applied mathematicians, they can then reconstruct it using agent-based computer simulations. This will then enable them to unravel how subtle changes in cell behaviour, driven by factors such as inherited disease or cancer, can affect the outcome of development and why. Thus, the resulting datasets become more meaningful descriptions of the observations as well as sets of rules to construct agent-based computer simulations of those observations. In this way, CBO takes a ‘cell-based approach’ [3], which views embryogenesis as the collective behaviour of a ‘colony’ of individual cells.
The extraction of cell behaviours from data, followed by the re-synthesis of the embryo as a computer simulation is already under way. At Inria (Roquencourt, France), a team led by Dirk Drasdo is using structural images to build simulations of liver regeneration following poisoning. At Inria (Montpellier, France), the VirtualPlants team headed by Christophe Godin has used detailed plant tissue images to build cell-level simulations of leaf initiation and vascular development in plants. In our own work here at CWI (Amsterdam), we are simulating the formation of blood vessel sprouts, e.g., during cancer neoangiogenesis, from the chemical and mechanical interactions between endothelial cells. As multicellular imaging datasets are merging with explanatory computer modelling, big data in biology is finally starting to really excite me.
Links:
Multicellular Modeling at CWI http://biomodel.project.cwi.nl
BioEmergences project: http://bioemergences.iscpif.fr
VirtualPlants team: http://www.inria.fr/en/teams/virtual-plants
Dirk Drasdo group: http://ms.izbi.uni-leipzig.de
CompuCell3D multicellular simulator: http://compucell3d.org
References:
[1] C. Castro-González et al.: “A Digital Framework to Build, Visualize and Analyze a Gene Expression Atlas with Cellular Resolution in Zebrafish Early Embryogenesis”, PLoS Comp. Biol., 10 (6), 2014, e1003670
[2] J.P. Sluka, J. P. et al .: “The Cell Behavior Ontology: Describing the Intrinsic Biological Behaviors of Real and Model Cells Seen as Active Agents”, Bioinformatics, 30(16), 2014, 2367-2374
[3] R.M.H. Merks and J.A. Glazier: “A Cell-Centered Approach to Developmental Biology”, Physica A, 352(1), 2005, 113–130.
Please contact:
Roeland Merks, CWI, The Netherlands
E-mail: