by Nicola Ferro and Gianmaria Silvello
Experimental evaluation of search engines produces scientific data that are highly valuable from both a research and financial point of view. They need to be interpreted and exploited over a large time-frame, and a crucial goal is to ensure their curation and enrichment via inter-linking with relevant resources in order to harness their full potential. To this end, we exploit the LOD paradigm for increasing experimental data discoverability, understandability and re-usability.
Experimental evaluation of multilingual and multimedia information access systems is a demanding activity that benefits from shared infrastructures and datasets that favour the adoption of common resources, allow for replication of the experiments, and foster comparison among state-of-the-art approaches. Therefore, experimental evaluation is carried out in large-scale evaluation campaigns at an international level, such as the Text REtrieval Conference (TREC) in the United States and the Conference and Labs of the Evaluation Forum (CLEF) in Europe.
Figure 1 shows the main phases of the experimental evaluation workflow, where the information space entailed by evaluation campaigns can be considered in the light of the “Data Information Knowledge and Wisdom” (DIKW) hierarchy, used as a model to organize the produced information resources [1]. Each phase is carried out by people with different roles and produces scientific data that need to be managed, curated, accessed and re-used.
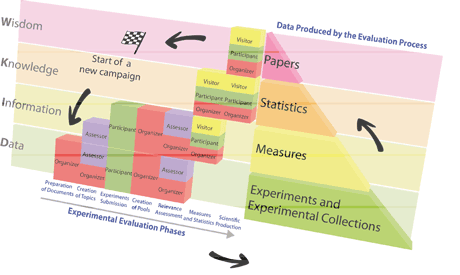
As a consequence, experimental evaluation has a big scientific and financial impact. From a scientific point of view, it has provided sizable improvements to key technologies, such as indexing, ranking, multilingual search, enterprise search, expert finding, and so on. From a financial point of view, it has been estimated that for every $1.00 invested in TREC, at least $3.35 to $5.07 in benefits accrued to researchers and industry, meaning that, for an overall investment in TREC of around 30 million dollars over 20 years, between 90 and 150 million dollars of benefits have been produced.
A crucial goal, therefore, is to ensure the best possible exploitation and interpretation of such valuable scientific data, possibly over large time spans. To this end, we have been modelling the experimental data and designing a software infrastructure to manage and curate them. This has led to the development of the DIRECT system [2]. Figure 2 reports the eight functional areas of the DIRECT conceptual schema [3], which allows the representation and management of the evaluation workflow along with the data produced, as reported in Figure 1. Not only do they cover the scientific data in a strict sense but they also address follow-up activities, such as data visualization and interaction and scientific and bibliographical production.
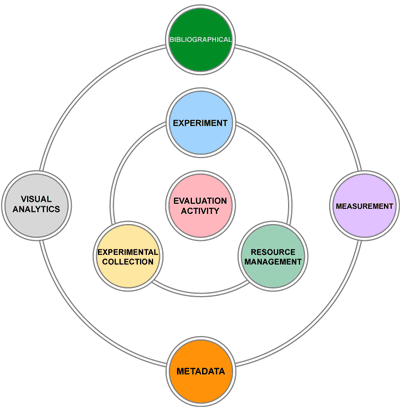
Some items of information - in particular, scientific data and literature - that are built upon these data grow and evolve over time. In order to make the information as easy as possible for users to discover, re-use and understand, thereby maximizing its potential, we need to ensure that the information is promptly curated, enriched, and updated with links to other relevant information resources.
In order to tackle these issues, we mapped the DIRECT conceptual model into an RDF schema for representing experimental data and exposing them as LOD on the Web. This enables a seamless integration of datasets produced by different international campaigns as well as the standardization of terms and concepts used to label data across research groups. Furthermore, we adopted automatic data enrichment methodologies focused on finding experts and topics in the produced scientific literature. These techniques also allow us to keep the experimental evaluation data continuously updated and linked in a timely manner to the LOD cloud.
Figure 3 shows a use-case of an RDF graph representing part of the experimental data enriched with the publications connected to them, the automatically defined expert profiles and the relationships with external concepts in the LOD cloud. The experimental data is shown in the lower part of Figure 3, where the CLEF campaign is connected to a track (AdHOC2008) composed by a task (AdHoc2008-TELCLEF); furthermore, we report an experiment (AdHoc-TEL-exp-001) submitted to that task. The relationships between a publication (Larson-CLEF2008), the experiment and the author (Ray R. Larson) are enriched by expertise topics (Logic Regression), expert profiles and connections to the LOD cloud.
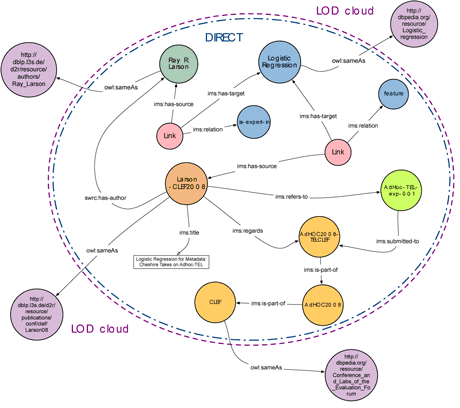
The connections between experiments and publications enable an explicit binding between the presentation of scientific results and the data actually used to achieve them. Furthermore, publications provide a description of the data, which increases their understandability and the potential for re-usability.
The author is also enriched with information about his or her expertise, and the publication is similarly enriched with information about its topic – logical regression in this case. Identifying, measuring, and representing expertise has the potential to encourage interaction and collaboration by constructing a web of connections between experts. Additionally, this information provides valuable insights to outsiders and novice members of a community.
Finally, the LOD approach allows new access points to the data to be defined; indeed, the expertise topics are connected to external resources belonging to DBPedia, and authors and contributions are connected to the DBLP linked open dataset allowing the experimental data to be easily discovered on the Web.
Future work will focus on the application of these semantic modelling and automatic enrichment techniques to other areas of the evaluation workflow. For example, the expert profiling and the topic extraction could be used to automatically improve and enhance the descriptions of the single experiments submitted to an evaluation campaign.
Links:
CLEF: http://www.clef-initiative.eu/
DIRECT: http://direct.dei.unipd.it/
PROMISE: http://www.promise-noe.eu/
TREC: http://trec.nist.gov/
References:
[1] M. Dussin and N. Ferro: “Managing the Knowledge Creation Process of Large-Scale Evaluation Campaigns”, in proc. of the 13th European Conference on Research and Advanced Technology for Digital Libraries (ECDL 2009), Springer LNCS 5714, 2009, dx.doi.org/10.1007/978-3-642-04346-8_8
[2] M. Agosti, G. M. Di Nunzio, N. Ferro: “The Importance of Scientific Data Curation for Evaluation Campaigns”, in proc. of the First Intl. DELOS Conference, Revised Selected Papers, Springer LNCS 4877, 2007, dx.doi.org/10.1007/978-3-540-77088-6_15
[3] M. Agosti, E. Di Buccio, N Ferro et al.: “DIRECTions: Design and Specifiation of an IR Evaluation Infrastructure”, in proc. of CLEF 2012, Springer LNCS 7488, dx.doi.org/10.1007/978-3-642-33247-0_11
Please contact:
Nicola Ferro, University of Padua, Italy
Tel: +39 049 827 7939
E-mail: