by Nicholas Loulloudes, George Pallis and Marios D. Dikaiakos
With the proliferation of wireless hardware that supports communication standards designed exclusively for the vehicular environment (IEEE 802.11p / WAVE), the wide deployment of Vehicular Networks (VANETs) is one step closer to realization. However, due to the inherent dynamics of mobility, the spatio-temporal topological features of such networks remain largely unknown, thereby putting at stake the quality of service provided by those intelligent applications for which VANETs were envisioned. The study of V2X communication dynamics from the aspect of Complex Network science can provide crucial insights to these features.
Our work stems from previous studies of large-scale, real-world systems (ie Internet topology, biological and social networks), which have led to important observations with significant influence [1,2]. During the design phase of applications such as safety message dissemination, congestion avoidance and infotainment that rely on V2X communications, certain key questions should be answered. For instance, when performing message routing, “What is the size and how well is the VANET connected in the arrow of time?”; when performing periodic 1-hop broadcasting, the question is “Does the topology support message spread with minimal rebroadcasts hence reducing latency, overhead and packet collisions?”; when performing multi-hop communication, “How far in the topology can packets travel?”; when the network is fragmented, “What are the properties of these clusters?”.
Using publicly available, highly realistic vehicular mobility traces [3] in a large-scale urban environment (~34 km2), we take per-second snapshots of the network over two hours, by considering the exact geographic position of ~53,000 nodes and the propensity for the establishment of an ad hoc wireless link given the communication range of V2X hardware. From accurate digital maps, we obtain the structure of the underlying road topology to establish a LOS/NLOS communication paradigm. Then we model the VANET topology as a graph, where vehicles correspond to vertices and links to edges. We analyze the structure and evolution of the communication graphs in variable traffic conditions and settings of V2X-enabled vehicle market penetration (P). Additionally, we extract the position of 7,000 signalized intersections in the area of study, which could potentially function as RSUs, and study their effects. Overall we analyse 436,000 graphs.
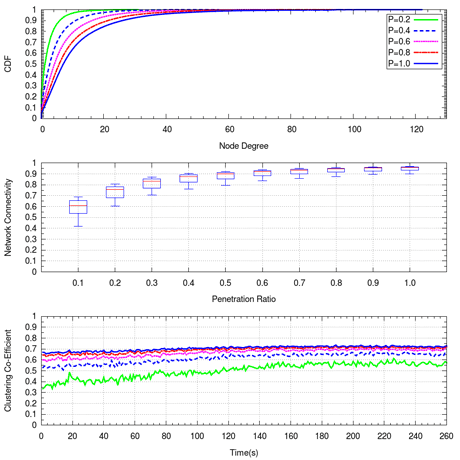
Our initial findings suggest that the VANET size (number of edges) grows linearly with increases in P. By examining the network connectivity (Figure 1a) we observe that even for values as low as P=10%, on average 62% of vehicles in the area of study were connected either with a direct or multi-hop link. This is counterintuitive since one would expect that in the case of a low P there would be a low probability that an encounter between two random vehicles could result in the establishment of a link. Furthermore, the geographical dispersion of the V2X-enabled vehicles, and consequently the distance between any established links, would prevent their fusion into multi-hop communication paths. When utilizing only 5% of the available RSUs, the minimum connectivity increases from 42% to 57%, showing that such stationary nodes can extend considerably the communication potential of vehicles. Studying the aggregate node degree distribution over the two hours (Figure 1b), we observe that VANETs are heterogeneous, where there can coexist several isolated vehicles and also others with over 100 neighbours. Nevertheless, we make an important observation in that the degree distribution does not follow a Power Law for any P, making VANETs not scale-free. Figure 2 depicts the empirical degree distribution (P=100%), which exhibits a cutoff at around 120 neighbours, in contrast to the power law fit that stretches on and on without a cutoff. Although at first sight vehicles with 120 neighbours look like “hubs”, they only appear at large network sizes and their links represent less than 1% of the network.
The network diameter exhibits very high variability, occasionally having shifts in excess of 20 hops, within only a few seconds. Moreover, the diameter follows the average degree of separation up to ~130 hops. The degree of separation increases almost proportionally to the geographic network size for all values of P, hence along with the fact that the degree distribution doesn’t follow a power-law, we are led to the conclusion that VANETs do not exhibit small-world properties.
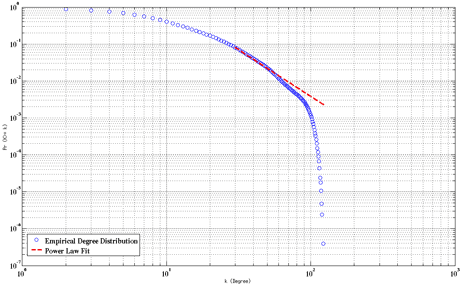
The VANET is partitioned in several clusters for all values of P. At low P it exhibits several small clusters, while high P favours the formation of at least one giant cluster (>0.1*network size) and a few smaller ones surrounding it. As P increases over 50% and a particular vehicle density threshold is reached, no new clusters are created. Newly arriving vehicles join one of the ~500 existing clusters. The average clustering co-efficient (Figure 1c) remains stable ~68% for P>=40%. This is a good indication of the connectivity of the nodes within the cluster, enabling message dissemination to a large audience with minimal broadcasts. Giant clusters such as that depicted in Figure 3 (in red), cover ~20% of the geographic area, however their shape and coverage changes rapidly in time. Effectively, given their strong internal connectivity, giant clusters enable the persistent dissemination and temporal maintenance of information over an extended geography.
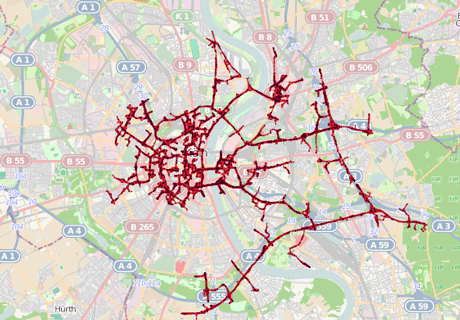
References:
[1] D.J. Watts, S.H. Strotgatz: “Collective Dynamics of Small-World Networks”, Nature 1998
[2] A. Barabasi, R. Albert, “Emergence of Scaling in Random Networks”, Science 1999
[3] TAPAS-Cologne dataset: http://sourceforge.net/apps/mediawiki/sumo/index.php?title=Data/Scenarios/TAPASCologne
Please contact:
Nicholas Loulloudes
Dept. Computer Science,
University of Cyprus
Tel: +357 22892676
E-mail: