by Evangeline Pollard, Fawzi Nashashibi and Paulo Resende
The purpose of the ABV project was to demonstrate the technical feasibility of fully automated driving at speeds below 50 km/h in urban and suburban areas with adequate infrastructure quality (no intersections, known road geometry and lane markings available). Researchers of Inria were in charge of the automation of an electrified Citröen C1 Ev’ie.
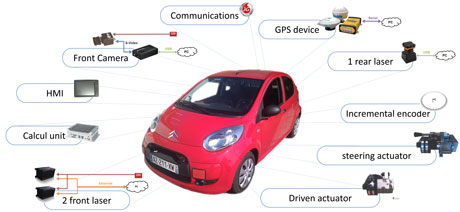
The Intelligent Transportation Systems (ITS) community has been focusing on vehicle automation since 1987, when the European Commission funded the 800 million Euros EC EUREKA Prometheus Project on autonomous vehicles. In this project, vehicles such as the Dickmann’s Mercedes-Benz were able to perform vision-based autonomous navigation on empty roads. Since this first European initiative, much work has been conducted in this area. Initiatives including the Cybercar (90’s), the PATH initiative (1997), the Darpa Grand Challenge (2004), the VisLab intercontinental autonomous challenge (2010) and Google car (2011) have contributed to this big challenge.
However, although more aspects of driving are being automated, full automation is still impossible in Europe for legal reasons. In order to create a legal framework for road automation and driving sharing, many projects, including the European project HAVEit, have tried to demonstrate its feasibility. The French Automatisation Basse Vitesse (ABV) project, standing for Low Speed Automation, differs from HAVEit by focusing on congested and heavy traffic in urban and suburban roads at speeds below 50 km/h and adding the fully automated driving capability to the automation spectrum.
By automatically following congested traffic, the ABV system relieves the human driver from performing monotonous tasks such as holding the brake pedal or rather risky maneuvers like changing lanes or simply keeping a safe distance from the vehicle in front. During fully automated driving inside the application zone, the human driver is still responsible for the vehicle, and is involved in the task of driving (eg activating a blinker to overtake) but with much less engagement. When reaching the end of the application zone the driver is required to take over the control of the vehicle. If the driver fails to do so, the vehicle will automatically stop.
The automation algorithms, following the classical scheme (1) perception (2) planning (3) control have been integrated into an electrified Citröen C1 Ev’ie and tested on several experiments on the Satory tracks (Versailles, France).
The Citröen C1 was equipped with sensors (odometers, lasers and frontal camera) in order to detect obstacles to avoid and determine which lane to follow, on-board computer to process data and make decisions, acceleration/brake and steering actuators (PARAVAN) to control the vehicle, and communication devices.
Perception issues are mainly divided into three axes. First the ego-localization allows the vehicle to know its position and orientation in the map (in the application zone). Position measurements coming from the GPS devices are fused with the velocity measurements coming from the odometers by using an Extended Kalman Filter (EKF). Obstacle detection and tracking is made with frontal and rear laser-scanners. Laser data are segmented using a Recursive Line Fitting algorithm to extract objects which are tracked with a Nearest Neighbour (NN) approach. Lane detection is made using the frontal camera. Images are transformed into bird’s-eye view images by Inverse Perspective Mapping, then a Canny detector is used to detect edges, which are filtered using a priori rules. Finally, extracted lines are filtered using a particle filtering.
Lane and obstacle detection are both used to plan the trajectory. A Lane Keeping System (LKS) allows the vehicle to stay in the middle of the current lane knowing the marking position. An Adaptive Cruise Control (ACC) is used to keep the vehicle at a safe distance from the vehicle in front. Relative velocity of the front obstacle is calculated by numerical derivation of the relative distance. An Obstacle Avoidance System (OAS) launches secured overtaking if activated by the driver. Finally, emergency braking can be activated by the driver or by the presence of an obstacle on the lane.
Control is achieved by automatically calculating the steering wheel’s angle and the velocity of the vehicle. Lateral control consists of following the reference line provided by the lane detection algorithm. For longitudinal control, a PID (proportional-integral-derivative controller) is used to maintain the velocity at the minimum velocity from among the following: The driver’s desired velocity; the legal speed limit; the speed limitation due to road geometry; and the front obstacle velocity.
Some work has been done on interactions between the system and the driver. A specific human-machine interface in the vehicle has been designed as a touch screen to let the driver choose the preferred speed and to initiate lane changes by activating the blinkers, if possible. A situation awareness component monitors the current state of the lanes (number of lanes, lane marking type, and current lane index), obstacles, ego vehicle state and current maneuver.
Numerous experiments have been conducted on the Satory tracks under several conditions. The vehicle has demonstrated its automation ability, as well as its driving sharing ability with the driver. Long term perspectives tend to ensure automation outside the application zone. Work is still in progress on the interaction between the driver and the car. There is no doubt that the future of automation lies in intelligent interaction, capable of assessing the driver’s, as well as the system’s, state.
Links:
https://www.youtube.com/watch?v=xsV8P7X4gvw
http://youtu.be/DoM06ho7JC0
http://www.projet-abv.fr/en/
References:
[1] H. Li and F. Nashashibi: “Robust real-time lane detection based on lane mark segment features and general a priori knowledge”, IEEE ICRB, 2011.
[2] P. Resende, et al.: “Low Speed Automation: technical feasibility of the driving sharing in urban area”, IEEE ITSC, 2013
Please contact:
Evangeline Pollard, Fawzi Nashashibi and Paulo Resende
Inria, France
E-mail: