by Stefano Basta, Fosca Giannotti, Giuseppe Manco, Dino Pedreschi and Laura Spinsanti
An effective audit strategy is a key success factor for 'a posteriori' fraud detection applications in fiscal and insurance domains. 'Sniper' is an auditing methodology with a rule-based system, which is capable of dealing with conflicting issues such as maximizing audit benefits, minimizing false-positive audit predictions and deterring probable future fraud.
Fraud detection represents a challenging issue in several application scenarios, and the automatic discovery of fraudulent behaviour is crucial in many real-life situations. In this context, the Value Added Tax (VAT) fraud detection scenario is receiving increasing interest for both its practical and theoretical issues. Like any tax, the VAT is open to fraud and evasion. There are several ways in which it can be abused, e.g. by underdeclaring sales or overdeclaring purchases. In addition, opportunities and incentives to fraud are provided by the credit mechanism that characterizes VAT: tax charged by a seller is available to the buyer as a credit against his liability on his own sales and, if in excess of the output tax due, refunded to him. Thus, fraudulent claims for credit and refunds are an extensive and problematic issue in fiscal fraud detection. From this perspective, a mathematical modelling methodology capable of producing a predictive analysis tool is of great significance. The tool should be able to identify the taxpayers with the highest probability of being VAT defrauders, in order to support the activity of planning and performing effective fiscal audits.
There are several issues that make the problem difficult to address. First, each government agency has a limited auditing capability, which severely restricts the amount of audited data available. In Italy for example, audits are performed on only 0.4% of the overall population of taxpayers who file a VAT refund request. This restriction inevitably raises a sample selection bias: while auditing is the only way to produce a training set upon which to devise models, auditors focus only on subjects who according to certain criteria seem particularly suspicious. As a consequence, the proportion of positive subjects (individuals who are actually defrauders) in the training set is vast compared with that in the overall population.
The limited auditing capability of a generic revenue agency poses severe constraints also in the design of the scoring system. Auditing is a time-consuming task involving several investigation and legal steps, and which ultimately requires a significant commitment of human resources. Hence, the scoring system should concentrate on a user-defined fixed number of individuals (representing the auditing capability of the agency), with high fraudulent likelihood and with a minimum false positive rate.
The situation is further exacerbated by the quest for a multi-purpose modelling methodology: in general, several objective functions characterize the fraud detection scenario, and a traditional classification scheme may fail in accomplishing such a multi-purpose task. Typically, experts are interested in scoring individuals according to three criteria:
- Proficiency: scoring and detection should not rely only on a binary decision boundary separating defrauders from non-defrauders. Rather, higher fraud amounts make defrauders more significant. For example, it is better to detect a defrauder whose fraud amounts to $1000 than one whose fraud amounts to $100.
- Equity: a weighting mechanism should highlight those cases where the fraud represents a significant proportion of the business volume. For example, an individual whose fraud amounts to $1000 and whose business volume is $100,000 is less interesting than an individual whose fraud amounts to $1000 but whose business volume is only $10,000.
- Efficiency: since the focus is on refunds, scoring and detection should be sensitive to total/partial frauds. For example, a subject claiming a VAT refund equal to $2000 and entitled to $1800 is less significant than a different subject claiming $200 who is entitled to nothing.
There are several mathematical tools based on machine learning and statistics, which can be adopted to address the above issues. Approaches based on the estimation of the underlying distribution and a direct modelling of the fraudulent behaviour exhibit shortcomings due to both the complexity of the domain under consideration and the presence of noise which prevents suitable model fitting. In general, supervised techniques (using a training set of known fraudulent cases) based on hybrid or cost-sensitive classification suffer from low interpretability, which makes them inadequate for the problem at hand. In addition, the aforementioned problem of sample selection bias makes it difficult to devise a proper training set. Recently, semi-supervised and unsupervised methods have been proposed to partially overcome these drawbacks. Unfortunately, these techniques fail to provide interpretable explanations of the 'outlierness' of a fraudster.
Rule-based approaches are preferable from a socio-economic point of view. Intelligible explanations of why individuals are scored as fraudulent are far more important than the scores themselves, as the former allow auditors to thoroughly investigate the behavioural mechanisms behind a fraud. Unfortunately, rule-based classifiers exhibit poor predictive accuracy when the underlying data distribution is inherently characterized by rarity and primary aspects of the concept being learnt are therefore infrequently observed.
In this context, we developed Sniper, a flexible methodology devised to accommodate all the above-mentioned issues in a unified framework. Sniper is an ensemble method that combines the best of several rule-based baseline classification tools, each of which addresses a specific problem from among those described above. The idea of the approach is to progressively learn a set of rules until all the above requirements are met. The approach is summarized in Figure 1.

Sniper devises a scoring function that associates an individual with a value representing degree of interest according to the proficiency, equity and efficiency parameters. Clearly, the training set of audited subjects allows the computation of such a function and its analytical evaluation over those known cases. Tuning the function allows different aspects of VAT fraud to be emphasized, from which baseline classification tools can then be trained in order to associate class labels to individuals according to their relevance to the aspect of interest. Figure 2a reports an example segmentation of the audited subjects on the basis of their relevance to the scoring function. Specifically, from the lighter to the darker-coloured slice, the figure reports the percentage of subjects in four segments. Conversely, Figure 2b reports the percentage of total amount of fraud associated to these segments.
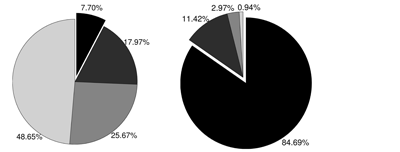
Baseline classifiers can thus be used to filter out rules that degrade the overall accuracy of the system. This is an iterative step, which selects the best subset of rules from that obtained by the baseline classifiers. The result is a final binary classifier capable of selecting subjects to be audited. Experimentally, Sniper has proven to be simple and effective, producing a prediction model that outperforms those models generated by traditional techniques.
Please contact:
Stefano Basta
ICAR CNR, Italy
E-mail: stefano.bastaicar.cnr.it
Fosca Giannotti
ISTI CNR, Italy
E-mail: fosca.giannottiisti.cnr.it
Giuseppe Manco
ICAR CNR, Italy
E-mail: giuseppe.mancoicar.cnr.it
Dino Pedreschi
Computer Science Dept. Univ. Pisa, Italy
E-mail: pedredi.unipi.it
Laura Spinsanti
ISTI CNR, Italy
E-mail: laura.spinsantiisti.cnr.it