by Annette Kik and Gunnar Klau
What does a disease like lymph cancer have in common with city heating? This unusual association popped up when CWI researcher Gunnar Klau discussed his work with a fellow researcher. Klau now exploits maths originally developed for optimizing heating networks to analyse data from cancer patients. His method appears to be faster and better than previous ones, and it is hoped that his results will help doctors in understanding the disease and in the end contribute to the quest for new and better medicines.
The types of cancer studied by Klau and his colleagues are called non-Hodgkin lymphomas - various malignant cancers originating in white blood cells and spread by the lymph node system. The disease is rather common, with several hundred patients in the Netherlands being diagnosed each year. In order to ensure a good prognosis and provide the best treatment, it is important to recognize the specific subtype of cancer that is involved. These cancer subtypes can be distinguished by a different kind of gene expression, ie the way genetic information is converted into functional products like proteins.
In molecular biology, the focus of research has recently shifted from decoding genetic sequences to analysing interactions that take place among genes and proteins. This is part of a new approach called systems biology. It is important to understand these interactions, since proteins do not function in isolation. They interact with each other and with other biomolecules to form molecular machines. These interactions are described by protein interaction networks. Huge amounts of experimental data on these networks are emerging from new high-throughput techniques like micro-arrays. Finding in a big network an active part (a subnetwork) that might contribute to the disease is like finding a needle in a haystack. When two thousand proteins are involved in a network, up to 4 × 106595 candidate subnetworks must be investigated.
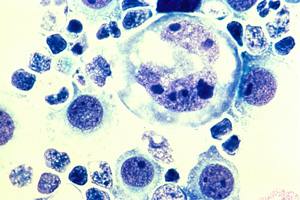
In spite of the apparent impossibility of this task, Gunnar Klau found a new method with which to tackle it. How does it work? Compare it to city heating," Klau explains. "You can have big houses and small houses, far away or close by. Big houses that are located close by are most profitable for the energy company. Due to their short lengths, the pipelines are not too expensive. On the other hand, a small house far away is not profitable and will probably not be connected to the city heating network at all. Traditional optimization methods only sought the big houses nearby and stopped calculating the moment they noticed a less favourable connection. However, it is possible that just beyond the small house a group of big, profitable houses can be found. Our method can find these kinds of groups."
In Klau's metaphor, a group of big houses stands for an active protein subnetwork. The problem of finding these subnetworks is very difficult: in mathematical terms it is known as NP complete. The lymphoma networks studied in this research count about 2000 nodes and 8000 connections. Klau used methods from discrete optimization to avoid looking at the exponential number of all subnetworks. First, he made a mathematical transformation to a known problem, the Prize Collecting Steiner Tree (PCST) problem. Then, together with statisticians from Würzburg, he developed a toolkit called 'Heinz'. Using data from medical experts, it took Heinz only a few minutes to enumerate possible interesting subnetworks!
Klau and his fellow researchers tested his system with known medical data, and found not only familiar subnetworks but even possible new interesting ones. The method is already better and faster than existing ones. "We still have to improve its accuracy and robustness," Klau says. "High-throughput biological data are extremely noisy, and the hidden subnetwork signals are quite weak."
In future, Klau wants to improve the model by integrating additional data sources to find biologically even more meaningful subnetworks. Klau: "This influences the mathematical model, because also the connections in the network will receive a score, based on co-expression of the two connected proteins. Then, the elegant transformation to the PCST-problem will not work anymore. We deal mathematically with a totally new problem, for which own theory has to be developed".
In the long term, it might be possible to analyse specific patient data faster with Klaus method. The pilot study has shown that it can distinguish between two types of lymphoma cancer. It might also recognize healthy patients from ill ones. "There is still a long way to go before this research can be applied in hospitals," Klau says. "However, I expect that it can help in studying network properties, identifying disease-related subnetworks, and network-based disease classifications. Ultimately, I hope the subnetworks computed with my mathematical methods will help to create new biological and medical knowledge which might lead to better cures."
For this research, Klau and his fellow researchers won the Outstanding Paper Award at the prestigious ISMB 2008 (16th Annual International Conference
Intelligent Systems for Molecular Biology) in Toronto, Canada. He cooperates closely with medical research partners like the Nederlands Kanker Instituut (NKI). "CWI finds it important to do this kind of interdisciplinary research," Klau says. We started a full Life Sciences Research cluster in 2009, to give this research a boost. I enjoy being part of this development.
Links:
http://homepages.cwi.nl/~klau/pubs/heinz_ISMB_2008.pdf
http://homepages.cwi.nl/~klau/
http://www.cwi.nl/lifesciences
Please contact:
Gunnar Klau
CWI, The Netherlands
Tel: +31 20 592 4117
E-mail: Gunnar.Klaucwi.nl