by Aiden R. Doherty and Alan F. Smeaton
We describe a wearable sensor technology that passively records 'lifelog' images and sensor readings of a wearer's daily life. The focus of our work is not on aggregating, collecting or networking data as in the usual application of sensors in the Sensor Web, but rather on detecting events of interest to the wearer from a multi-sensor standalone device. These events of interest provide effective cues to allow people to more easily access their autobiographical memories. Early research indicates this technology may be potentially helpful for sufferers of neurodegenerative diseases such as Alzheimer's.
Sensors and sensing technology are everywhere, and this issue of ERCIM News contains many examples of sensors networked together for some greater purpose. Mostly, people deploy sensors and then gather the readings together and address issues like networking, calibration, sensor fusion and sensor event detection. The general trend is towards networking sensors into the Sensor Web, but this isn't the only way of using them. Sensors can be used in small groupings on standalone devices that gather and process information and report back not sensor readings, but major semantic events. In this article we describe one such sensor technology which is simple and cheap to manufacture, but can empower an individual to reflect on their past behaviour and memories.
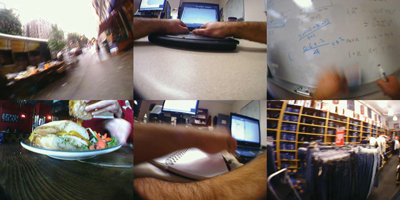
Lifelogging is the term used to describe the recording of different aspects of your daily life, in digital form, for your own exclusive personal use. It can take many forms, such as an application running on your mobile phone that 'logs' all your phone calls. One particularly interesting device is the SenseCam, a camera that is worn around the neck and automatically captures thousands of images of the wearer's life every day. It has a range of in-built sensors for monitoring the wearer's environment, detecting movement, ambient temperature, passive infrared information (ie body heat) and light intensity.
Preliminary studies indicate that information gathered by the SenseCam is potentially useful as a memory aid to recall autobiographical memories. Research in the field of cognitive neuropsychology has established that 'cued recall' is better than 'free recall'. The closer a cue is to how an actual memory was encoded, the better memory retrieval is. Other studies indicate that autobiographical memories tend to be strongly encoded in a visual manner in the brain. The SenseCam records pictures from the viewpoint of the user, making it able to provide visual cues of our past that are very close to how the original memories/experiences were encoded in the brain.
Even though SenseCam images provide strong memory cues, there exists a substantial problem in effectively managing the overwhelming volume of images generated by this device approximately 650 000 images per year are captured. Within the CLARITY centre at Dublin City University, we have developed a suite of functions applied to SenseCam data that automatically provide effective digital memory retrieval cues. We structure our processing into a number of logical steps that exploit various characteristics of the human memory system.
1. Firstly, we intelligently segment sequences of images into distinct events such as having breakfast, working on a computer etc. This is achieved very quickly using on-board environmental sensor values.
2. Given that human memory stores information associatively, we provide users with automated search functions to find events similar to a given event, eg "show me other times when I was at the park". By intelligently representing events through the fusion of image descriptions and the in-built sensor values, we found that users can find events related to any given 'query event'.
3. Given that the human memory more strongly encodes distinctive memories, we automatically identify events that are more visually unique among those recorded by a wearer. We have found that it is effective to combine the automated detection of faces (to indicate social engagement) with detecting how visually novel each event is.
4. As human memory is known to store items associatively, it is useful to augment individuals' SenseCam events with images (or videos) from external sources, eg to better remember a trip to the Eiffel Tower by viewing pictures of the tower uploaded by others to the Internet. Using GPS information, and after some intelligent automated processing, we can automatically find relevant supplementary images and videos from Internet sites such as Flickr and YouTube.
Our technology for autobiographical memory capture and management has been deployed not only within our research centre in Dublin, but also in numerous cognitive psychology research groups in Europe and North America, and uses sensors and a camera to gather information as part of a lifelog. These include Universities of Toronto, Tampere, Illinois, Utrecht and CWI in Amsterdam. Our approach does not conform to the common model of a sensor network composed of inter-connected sensors with live, real-time streaming data. This is because the demands of lifelogging are for post-event reflective retrieval rather than real time, meaning live inter-connectivity with other sensor nodes is not as vital as in other Sensor Web technologies.
Link:
http://www.cdvp.dcu.ie/SenseCam/
Please contact:
Aiden R. Doherty, Alan F. Smeaton
CLARITY Centre for Sensor Web Technologies, Dublin City University, Ireland
E-mail: adohertycomputing.dcu.ie, alan.smeaton
dcu.ie