by Eric Pauwels, Albert Salah and Paul de Zeeuw
Sensor networks are increasingly finding their way into our living environments, where they perform a variety of tasks like surveillance, safety or resource monitoring. Progress in standardization and communication protocols has made it possible to communicate and exchange data in an ad hoc fashion, thus creating extended and heterogeneous multimodal sensor networks. CWI is looking at ways to automatically propagate semantic information across sensor modalities.
Wireless sensors are deployed in a growing number of applications where they perform a wide variety of tasks. Although this has considerable economic and social advantages, it seems likely that even greater benefits can be gained once heterogeneous sets of individual sensors are able to communicate and link up into larger multimodal sensor (inter)networks. We expect that the network's performance will become more robust when information from multiple sources is integrated. In addition, networks could become smarter for at least two reasons: sensors that produce highly reliable output can be used to provide on-the-fly 'ground truth' for the training of other sensors within the network, and correlations among sensed events could bootstrap the automatic propagation of semantic information across sensors or modalities.
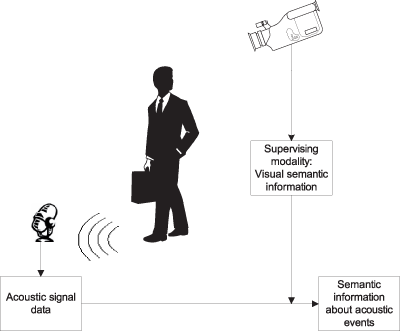
The implementation of our vision requires two conditions to be met. Firstly, sensors should come equipped with an open interface through which their output data and all relevant metadata can be made available for third party applications. Secondly, sensor networks need to be endowed with a learning mechanism that shifts the burden of supervision from humans to machines. Indeed, an additional layer of intelligence on top of the communication protocols will enable sensors to advertise their own capabilities, discover complementary services available on the network and orchestrate them into more powerful applications that meet high-level specifications set by human supervisors. This can be achieved more efficiently if the capabilities of the different components can be described in both human- and machine-readable form. It will then be possible for individual sensors to relate their own objectives and capabilities to human-defined goals (eg minimize energy consumption without sacrificing comfort) or available knowledge, both of which are usually expressed in terms of high-level semantics.
Granted, the linking of low-level sensor data to high-level semantic concepts remains a formidable problem, but we contend that the complementarity inherent in the different sensing spectra supported by such a network might actually alleviate the problem. The basic idea is simple: if particular sensor data can be linked to specific semantic notions, then it can be hypothesized that strongly correlated data picked up by complementary sensors (or modalities) are linked to semantically related concepts. A simple example will clarify the issues at hand: imagine a camera network on a factory floor that has been programmed to identify persons using face recognition, and to determine whether or not they are walking, say for safety reasons. If the same factory is also equipped with open microphones that monitor ambient noise, then an intelligent supervision system might pick up the strong correlation between walking people as observed by the camera network and rhythmic background sounds as detected by the microphones.
By mining general knowledge databases, the system might then be able to conclude that the observed rhythmic audio output corresponds to the sound of footsteps and add this snippet of semantic information to its knowledge database. In essence, the system succeeded in using available high-level information (the visual recognition of walking people) to bridge the semantic gap for an unrelated sensor (audio). By accumulating the information gleaned from such incremental advances, we contend that it will be possible to gradually - but largely automatically - extend the system's knowledge database linking low-level observed sensor data to high-level semantic notions.
To explore the viability of this idea we have conducted a number of simple experiments in which we used the Internet as a general knowledge database. For instance, referring to the above scenario we submitted the paired search terms walking (as the camera has been programmed to detect this behaviour) and sound (through the use of standards such as SensorML, each sensor can communicate the modality of its output) into a search engine and analysed the response. By restricting attention to meaningful words that occur frequently (both in terms of number per page and number of unique pages), we end up with a sorted list that suggests a link between the audio data and a list of semantic concepts including music, video, gait, work and footsteps. In a final step this list is further whittled down by checking each of these suggestions against an ontology to determine their semantic distance to the original concept (walking). By restricting attention to the most similar concepts, it transpires that it is highly likely the recorder audio is related to either footsteps, gait or music, all of which make sense. These results hint at the possibility of automatically extending semantic notions across modalities, thus leading to more robust and intelligent networks.
Link:
http://www.cwi.nl/pna4
Please contact:
Eric Pauwels
CWI, The Netherlands
Tel:+31 20 592 4225
E-mail: Eric.Pauwelscwi.nl