by Thiemo Voigt, Nicolas Tsiftes and Zhitao He
Sensor networks enable remote monitoring of natural environments such as glaciers, volcanoes and bodies of water. Within the project 'Sensor Networks to Monitor Marine Environment with Particular Focus on Climate Changes', SICS and partners are designing and implementing flexible, reprogrammable sensor network solutions suitable for monitoring the marine environment with high resolution in time and space.
Carrying out marine research requires that studies be undertaken in remote environments such as the Baltic Sea. However, marine environmental monitoring is expensive: the cost of operation at sea includes at least 10 000-15 000 per day for the use of a lar ge research vessel, plus the cost of laboratory technicians, analytical instrumentation and logistics. As a result, the marine environment is poorly monitored. For example, in the Baltic Sea's Bothnian Bay, the nine fixed stations are visited only eight times a year.
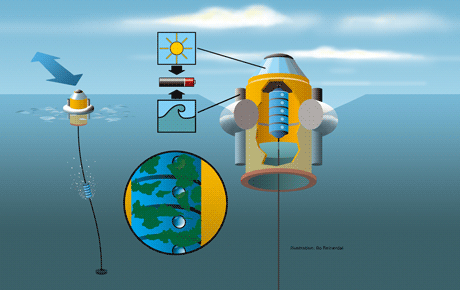
Sensor networks mean that marine data collection can be undertaken in a much more cost-efficient fashion. Within the multi-disciplinary project 'Sensor Networks to Monitor Marine Environment with Particular Focus on Climate Changes', SICS and partners have designed an advanced water monitoring system (Figure 1). Our system features a diving unit, consisting of a bin containing several sensors connected to one sensor node. The diving unit moves up and down an anchor line. In this way we are able to take measurements at any depth using only one set of sensors. A pressure sensor determines the depth at which measurements are being recorded. When the diving unit is not in the water taking measurements, it is parked in a garage that is part of our buoy construction. By parking the diving unit in the garage, we expect to eliminate fouling, ie the accumulation of material on hard surfaces in aquatic environments. We first intended to use an oil-filled garage, but found that this affected the sensor readings. Initial experiments with an air-filled garage have shown that this solution also prevents fouling.
Our system is driven by rechargeable batteries. Previous projects have had problems with solar power. Our project partner Uppsala University therefore designed a wave energy generator to obtain electric power from vertical wave movements. As a ring of stacked magnets floats up and down along a rod wound with metal coils, an alternating voltage is induced across the two ends of the coil. This is further rectified and filtered to provide a constant charging voltage to the batteries.
Our system is designed to cope with water depths of around 100m, meaning the number of measurements taken during one dive can be significant. Storage is thus required for the large volumes of measurement data: not only those collected by the diving unit but also from light and acceleration sensors placed on the top of the buoy. System data also needs to be saved; for example, the energy produced by the wave energy generator as well as the available energy. The sheer amount of data exceeds the limited RAM available in sensor nodes. Moreover, to handle the different data types smoothly, we use Contiki's Coffee file system to store data. The data is transferred onshore using GPRS (General Packet Radio Service), and this is too energy-consuming a task to be performed after every dive. We have therefore ported Coffee to operate on SD cards that enable cheap mass storage.
Due to the high energy consumption of the GPRS unit as well as the per-byte cost of the transferred data, we compress the data before sending it. Previous work has shown that compression is much cheaper than communication for typical sensor nodes with low-power radios. The same is true for the more power-hungry GPRS communication. To this end, we have designed a new compression algorithm called SB-ZIP that is more efficient than state-of-the-art compression algorithms. For example, SB-ZIP compresses 4.5 MB of acceleration data measured on the buoy to only 1.5 MB, whereas the well-known S-LZW algorithm is only able to reduce it to slightly over 3 MB.
SICS is running this project in collaboration with Umeå Marine Sciences Centre, Uppsala University and SMHI, the Swedish Meteorological and Hydrological Institute. At the conclusion of the project in late 2009, we expect to have deployed around ten buoys at places relevant for marine monitoring in the Baltic Sea.
Link:
http://www.sics.se/node/1361
Please contact:
Thiemo Voigt, SICS, Sweden
Tel: +46 8 633 1598
E-mail: thiemoics.se