by Christian Bauckhage, Markus Ketterl and Ulrich Nütten
LEARNMINER is an interactive social eLearning platform that integrates advanced analytics and linked data processing to assist users in structuring course material. Tools for analyzing crowd behaviour allow instructors to identify overly simple or difficult material and provide means for planning deadlines or tests. LEARNMINER allows for mobile access and provides interactive visualization of learning material and progress.
The interdisciplinary field of Web science studies technical and sociological aspects of the Web. Given that the Web is the largest human information construct in history whose rate of adoption and impact on society are unparalleled, Web science aims to understand the Web in order to engineer its future and to ensure its social benefit [1]. The research project SoFWIReD (Southampton Fraunhofer Web Science, Internet Research & Development), funded by the Fraunhofer ICON initiative, is a collaboration between the University of Southampton and Fraunhofer FOKUS and Fraunhofer IAIS. It involves web and data scientists with expertise in developing web platforms for data and knowledge driven processing that incorporate aspects of collective intelligence.
One of the platforms developed in SoFWIReD is LERANMINER, an eLearning solution that aims to simplify and optimize the process of learning and training on the job. It integrates social media features, linked Open Data processing, document analysis, and predictive analytics.
Confronted with large repositories of learning material, users may struggle to decide which item to work with next since this decision requires knowledge as to the content of the whole repository. LEARNMINER assists users by automatically determining document semantics and interrelations. It structures learning resources and displays them as a dynamic document graph which evolves with the progress of individual users as well as crowds of users. The knowledge graph thus helps users to prioritize study according to their own needs as well taking into account the experience of the overall population. In other words, the wisdom of the crowd is harnessed to facilitate the task of the individual. Analytics tools help instructors to track user progress or to predict when learning tasks will be accomplished. This in turn facilitates properly defined deadlines, homework, and tests.
LEARNMINER provides a semantic search engine for users to navigate through a repository of documents. Using semantic text mining [2], queries are matched to semantic categories extracted from a given repository. Search results are ranked according to their semantic similarity to a query and displayed together with the knowledge graph to allow users to contextualize material.
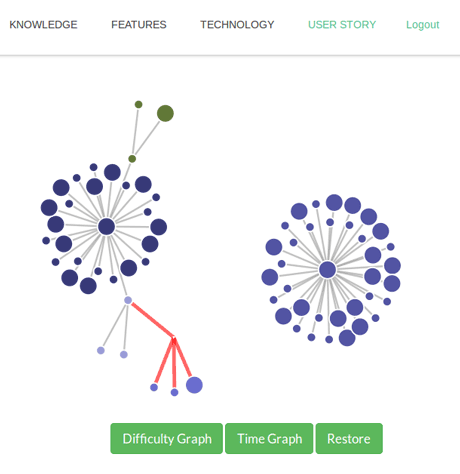
Figure 1: Learn path visualization of a LEARNMINER knowledge graph.
When displaying the knowledge graph, users have different options. In the basic view, documents are structured according semantic similarities and shown as interlinked nodes. Node colours reflect subject areas and the most general document of a subject area is displayed as the central node of a subgraph. Node sizes are used to express document sizes (e.g., measured in text length); the bigger a node, the more time users are expected to devote to the corresponding document.
When displayed as a difficulty graph, the knowledge graph is organized according to how the overall user population performs on tests created for each document. The more difficult a document the farther away it is from the cluster centre. The time graph display organizes the knowledge graph such that it reflects the time that the user has spent working with a document. Documents close to the centre of the graph indicate material a user has (almost) completed, for example, by reading (almost) all pages of a document and taking corresponding tests. Documents farther from the centre indicate material which still requires more time to completion. Finally, the learn path display provides suggestions about how to continue studies by drawing a personalized path through the space of available resources. That is, this representation guides users based on their experience, background knowledge, and learning progress. While users are working LEARNIMER, their learn paths will adapt and individual and collective progress and success (e.g., measured in terms of grades obtained) are parameters used to compute suggestions for further reading.
LEARNMINER is currently employed in a corporate training environment, and users report that they especially appreciate its social features (e.g., support for study groups). By design, the platform is interoperable and can efficiently index and structure learning repositories for a wide range of subject areas.
References:
[1] J. Hendler, et al.: “Web science: an interdisciplinary approach to understanding the Web,” Communications of the ACM, vol. 51, no. 7, pp. 60-69, 2008.
[2] F. Metze, C. Bauckhage, and T. Alpcan: “Social and Expert Research in Online Communities”, in Semantic Computing , P. Sheu, et al. (eds), Wiley, 2010.
Please contact:
Ulrich Nütten, Fraunhofer IAIS, Germany
Tel: +49-2241-142915
E-mail: