DesParO, an interactive environment for design parameter optimization, was developed at the Fraunhofer Institute for Algorithms and Scientific Computing SCAI. Its operation involves the initial sampling of a space of design variables by a limited number of simulations using Design of Experiments techniques. On the basis of these samples, DesParO constructs a nonlinear metamodel with radial basis functions. The user is then able to explore interactively the whole space of design variables and to find the optimal region with respect to multiple design objectives.
DesParO allows design problems to be interactively explored by user-driven continuous change of design variables and instant evaluation of design objectives. Constraints to the design objectives can be applied by setting upper/lower boundaries on corresponding axes. For example, an operation such as the minimization of a single objective can be achieved by shifting the objective's upper boundary towards the minimum. The availability of solutions is then immediately visible as the appearance of 'green islands' on the axes of design variables and design objectives.
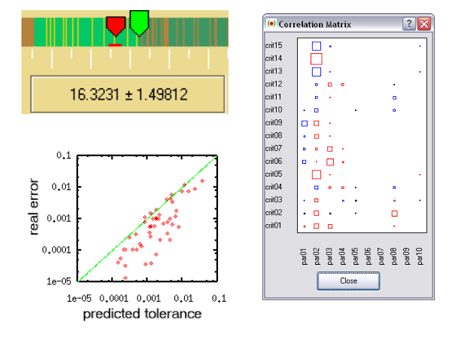
Successful optimization with a metamodel is possible only if its tolerances are taken into account. An important feature of DesParO is therefore robust tolerance prediction: the metamodel predicts not only the value of the design objective, but also the tolerance limits on the objective. For noisy objectives this allows constraints including the tolerance to be satisfied in a safe manner, and robust optimal design to be obtained with guaranteed 3sigma confidence.
In estimating the tolerance, the following cross-validation procedure is used. In the dataset composed of simulation results, one of the points is removed and the metamodel interpolates simulation results to this point. The obtained interpolated result is compared with the real simulation result, and their difference yields the tolerance of the metamodel at the given datapoint.
Due to the interpolation property, the metamodel exactly reproduces the values at those datapoints, which are included in the dataset. As a result, the tolerance at the datapoint equals the deviation of the metamodel when this point is removed from the dataset. For intermediate points that do not belong to the dataset, the predicted tolerance is defined analogously as the deviation of the metamodel when each of the datapoints is removed, combined by a cumulative criterion. Using fast update methods, we are able to evaluate the described tolerance characteristics in real time, together with the values of criteria.
DesParO also features global sensitivity analysis. A pattern of interdependencies between the optimization criteria and the design variables is automatically recognized and represented as an easy-to-read colour-coded diagram. The diagram indicates the most influencing design variables and the most sensitive optimization criteria, and also shows a sign of dependency: increase (red), decrease (blue) or non-monotony (black).
While the general purpose of optimization is to find an optimal design (eg the minimal value of a design objective), scatter analysis can reveal a robust design (minimal error of design objective). DesParO unifies these two capabilities and allows users to find the robust optimal design, where both the values and the errors of design objectives are optimal.
Additionally DesParO provides interpolation of bulky data, such as FEM data files containing the results of numerical simulation, for the user-specified values of design variables. This allows users to visualize a full solution immediately and to inspect the resulting optimal design in detail.
DesParO not only predicts tolerances of single criteria values but also estimates the uncertainties of distributed values stored in FEM data files. The estimated uncertainty is a good measure of scatter or essential non-linearity in such bulky data. Designs with small error are predictable and robust, while the parts with high uncertainty have unpredictable behaviour.
DesParO has been successfully applied to the solution of several real-life optimization problems; in particular, the constrained car design optimization at Volkswagen AG. The goal is to minimize the total mass of the vehicle by varying the thicknesses of fifteen car body components while simultaneously targeting constraints on eleven additional optimization criteria related to safety and comfort characteristics. Optimization with DesParO has allowed to achieve a significant mass reduction while satisfying all constraints. Robustness of the design is also evaluated using computation of distributed uncertainties in DesParO.
Link:
http://www.scai.fraunhofer.de/desparo.html?&L=1
Please contact:
Daniela Steffes-lai
Fraunhofer Institute for Algorithms and Scientific Computing SCAI, Germany
Tel: +49 221 470 6089
E-mail: daniela.steffes-laiscai.fraunhofer.de