by Sébastien Piérard, Samir Azrour, Rémy Phan-Ba and Marc Van Droogenbroeck
Gait observation and analysis can provide invaluable information about an individual [1]. Studies that have interpreted gait using traditional imaging devices have demonstrated that it is difficult to make reliable measurements with colour cameras. GAIMS, our new system resulting from a multidisciplinary project born from collaboration between engineers and neurologists, aims at developing non-intrusive and reliable tools to provide quantitative measures of gait and interpretations of the acquired data. Following a current trend in imaging, it takes advantage of imaging sensors that measure distance instead of colour. While its principles are general, GAIMS is currently used for the diagnosis of multiple sclerosis (MS) and the continued evaluation of disease progression [2]. It is the first available system to fully satisfy the clinical routine and its associated constraints.
{jcomments on}Gait is a complex process involving neurology, mechanics and muscle. Gait is unique to the individual [1], and depends on many factors including: age, sex, weight, height, the presence of disease, and medication. Because the gait is so dependent on these factors, it is possible to infer some information about an individual based on his gait.
While colour images have been used intensively for gait analysis and recognition, they are inadequate for deriving precise quantitative measurements and objective information about the walking person. Therefore, at the University of Liège, we have developed GAIMS, a non-intrusive gait analysis and imaging system based primarily on range laser sensors. In our setup, several range laser scanners, which can be seen as 3D cameras reduced to one line, are combined to reconstruct a horizontal slice of the scene, at a height of 15 cm above the floor. While we hope to be able to build denser 3D information utilizing all the information provided by 3D cameras in the future, in its current form GAIMS is able to reconstruct the trajectories of both feet, and to provide derived gait measures.
GAIMS has numerous advantages. It provides a range of information, such as the location of the feet, the walking speed, the spacing between the feet, the double support duration, etc, which can be combined with images to form a bridge between the observation and interpretation of complicated scenarios such as gait analysis. Knowledge about the location and dynamics of individuals within the scene is obtained without the need for the subjects to wear any sensor, marker, or reflector. GAIMS is therefore a valuable tool in applications such as trajectory analysis for behaviour interpretation or clinical evaluation. In addition, GAIMS operates over a large area (about 10m wide) and it is almost insensitive to ambient lighting.
For the interpretation, we have developed, on top of our system, a set of intelligent tools dedicated to the specific data type, that combine filtering, tracking, machine learning, and some heuristics. As the relationship between the factors influencing the gait and the gait itself is complex and still largely unknown, gait interpretation is a delicate task: an expert is usually able to interpret the observations, but rarely able to explain how he proceeds.
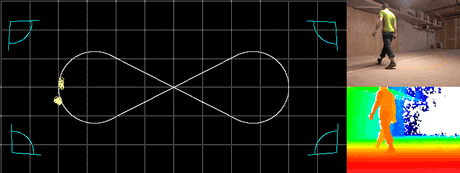
GAIMS can help with the diagnosis of multiple sclerosis (MS) and for the continued evaluation of the disease’s progression [2]. The ambulation impairments appear in the early stages of the disease and the magnitude of gait modification is a good indicator of disease activity. The current standardized tests used to assess gait in MS are still based largely on a measure of speed, and a simple stop watch is often the sole measuring instrument used by the neurologist. This is too limiting. GAIMS is the first powerful system that fully satisfies the clinical routine constraints and is compatible with existing standardized tests. Its effectiveness has been shown through a threefold validation procedure: (1) GAIMS has been demonstrated to detect subtle (intra-subject) gait alterations and to be more effective than a stop watch in detecting gait abnormalities. (2) It is possible to differentiate between healthy individuals and MS patients based on gait characteristics measured by GAIMS. (3) GAIMS can also measure significant differences between MS patients with different disease states.
While it has been successfully used for multiple sclerosis analysis, GAIMS is not limited to medical applications. For example, we have used it in conjunction with 3D cameras and projectors to create a 3D immersive and interactive system that gives the illusion of a 3D virtual world all around the user [3]. GAIMS has the power to tell you precisely where the user is located. This is useful for real time scene interpretation and interactive applications.
References:
[1] N. Boulgouris, D. Hatzinakos, and K. Plataniotis: “Gait recognition: a challenging signal processing technology for biometric identification”, IEEE Signal Processing Magazine, 22(6):78– 90, Nov. 2005
[2] S. Belachew et al: “Multimodal evaluation of gait and stride dynamics in relapsing and progressive forms of multiple sclerosis”, in proc. of the Belgian Royal Academies of Medecine, 1:66–69, 2012
[3] S. Piérard et al: “I-see-3D! An interactive and immersive system that dynamically adapts 2D projections to the location of a user’s eyes”, in proc. of IC3D, Liège, Belgium, December 2012.
Please contact:
Sébastien Piérard
INTELSIG Laboratory, Montefiore Institute, University of Liège, Belgium
E-mail: