Notwithstanding this progress, a major obstacle for further improvement of climate and weather models is the current treatment of sub-grid-scale processes in these models. Currently, weather prediction models covering the entire globe have grid spacings of at least 10-20km; grid spacings in climate models are about 100km or more. Physical and dynamical processes with spatial scales below this grid scale cannot be resolved explicitly in numerical models, even though they can be very important for the behaviour of atmosphere and climate. Making steps forward in the treatment of these sub-grid-scale processes is paramount to model improvement and thereby to more accurate forecasts and climate studies.
A prime example of an important yet small-scale process is convection: the vertical motion of (moist) air, intimately related to cloud formation and precipitation. Numerical models that can resolve convection and cloud dynamics need grid spacings of less than one kilometre. Because such high resolution is out of reach for global models, the impact of convection and clouds on resolved model variables must be represented in one way or another. These representations are called parameterizations. Because of the importance of convection, in particular for the tropical atmosphere, the quality of convection parameterizations has a significant impact on the overall accuracy of weather and climate models.
Operational weather and climate models employ a suite of parameterizations, thereby aiming to represent the effect of a collection of unresolved processes. Most of the parameterization schemes currently used are deterministic in nature: each state of the resolved model variables gives a single, unique outcome of the parameterization scheme. For example, air temperature and humidity are resolved explicitly at dozens of vertical levels throughout the atmosphere. One class of convection parameterization schemes takes the vertical profiles of humidity and temperature in a vertical column of the model and determines what convection and precipitation (if any) occurs. If the same constellation of vertical profiles occurs twice, the parameterized convection and precipitation will be the same too.
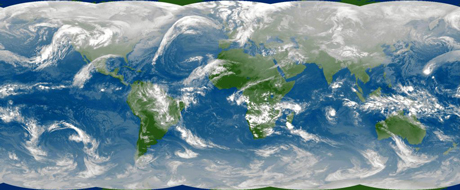
In recent years, researchers have come to realize the limitations of this deterministic approach. Unresolved processes may well have their own dynamics and need not be slaved to the resolved model variables. Even if one is able to calculate correctly the mean impact of the unresolved processes, neglecting their inherent variability is detrimental to the quality of the model simulations. In terms of the example just given: while the parameterization scheme may assign the correct mean convection and precipitation to given vertical profiles, in reality there may be significant deviations from this mean response. Interestingly, such deviations can become more important if the model resolution increases: a higher resolution means fewer convective cells in each vertical model column, so that the sample mean of the convective cells in a single column should vary more between columns.
In order to account for the inherent variability of many sub-grid-scale processes, researchers have started to look at alternative approaches to parameterization. One approach that has drawn a lot of research interest concerns stochastic parameterizations: approaches that carry elements of randomness in them. The basic idea is to let chance (in fact, a random number generator) choose from a range of possible responses at each time step of a simulation, rather than to use some kind of mean response of the sub-grid-scale process.
Stochastic parameterizations can take many shapes and formulations. For example, there are several possible answers to each of the following questions: (i) what class of random processes or stochastic models should be used? (ii) how can the correct parameters of such a model be obtained (thereby determining, for example, the distribution of possible sub-grid-scale responses)? and (iii) how should the stochastic model(s) for the sub-grid-scale responses interact with the model for the large-scale (resolved) variables?
Recent work carried out at CWI in collaboration with New York University employs conditional Markov chains to formulate a stochastic parameterization scheme. The sub-grid-scale responses are modelled as Markov chains, implying that there is a finite number of possible responses. The responses evolve in time by jumping randomly between different possibilities, in a way that is consistent with a set of jump probabilities derived from data of the sub-grid-scale processes. The jump probabilities depend on the (time-evolving) state of the resolved variables, hence the term 'conditional Markov chain'. This approach was successfully implemented in a test model environment, resulting in more faithful model statistics, improved forecast skill and better ensemble forecasting properties (so-called ensemble spread). Future work will focus on implementation in models of higher complexity.
Links:
http://www.cwi.nl/~dtc/
Please contact:
Daan Crommelin
CWI, The Netherlands
Tel: +31 20 592 4194
E-mail: Daan.Crommelincwi.nl